Statistically Enhanced Learning: a Formalization Framework of Feature Extraction Techniques
Conference
64th ISI World Statistics Congress
Format: CPS Poster
Keywords: extraction, feature, learning, machine learning, statistics
Session: CPS Posters-02
Monday 17 July 4 p.m. - 5:20 p.m. (Canada/Eastern)
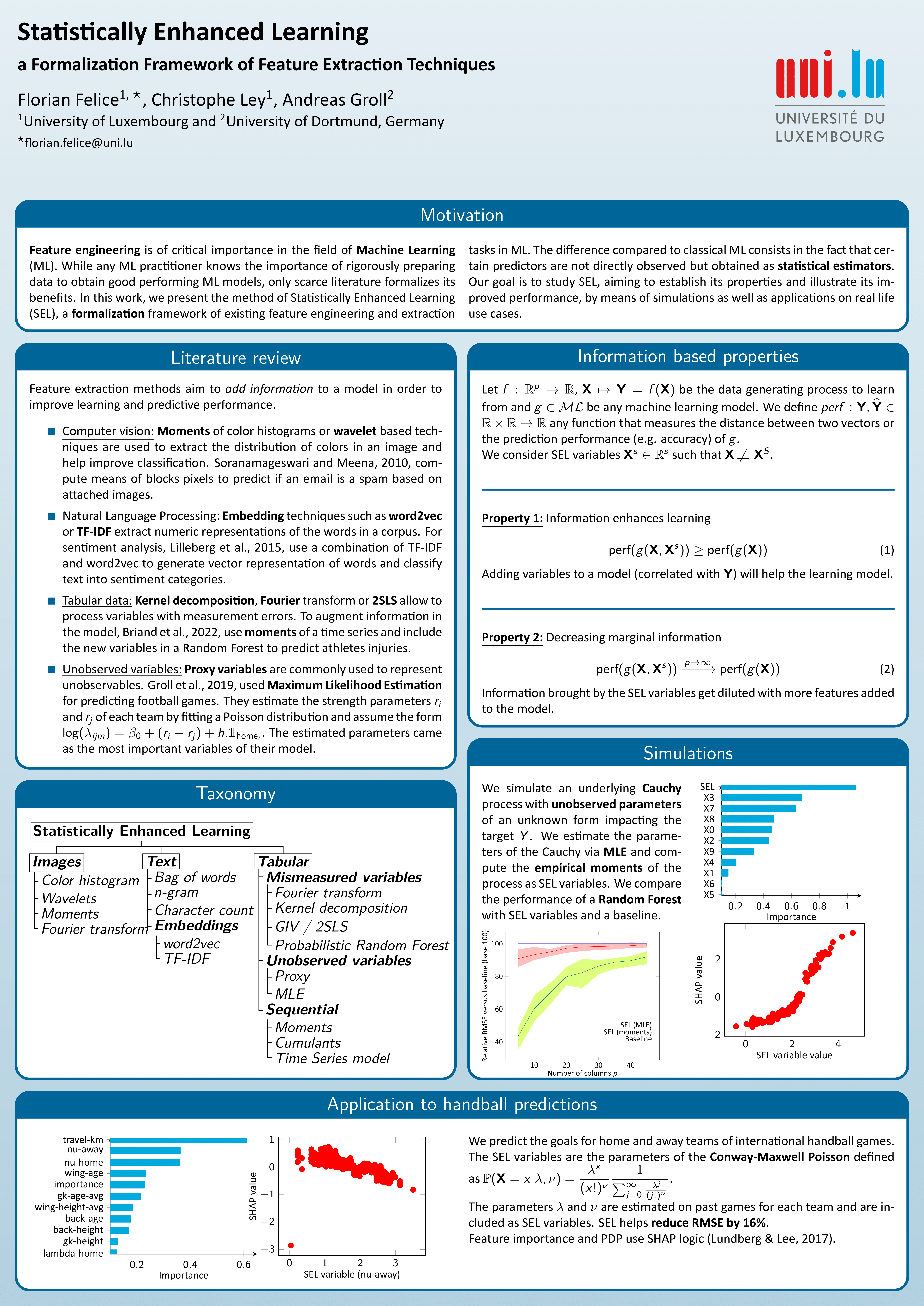
Abstract
In the field of Machine Learning (ML) the preparation of the data is often considered more important than the model itself. ML students are usually taught that 80% of the workload on an ML project is preparing the data.
The 20% remaining are left for modelling. While this is crucial to get a reliable model with high performance, there is little literature covering data preparation and its benefit to models.
In this work, we will present the method of Statistically Enhanced Learning (SEL), a generalization and formalization framework of existing data preparation steps in ML that rely on statistical estimators.
In SEL, learning, as the general term, stands for the large spectrum of data driven learning techniques (from classical statistical to advanced deep learning models).
The term statistical refers to features that are generated using observed variables in the practitioner's data set.
They can range from basic estimators of mean or variance to maximum likelihood based quantities.
The difference with classical ML consists in the fact that certain predictors are not directly observed but obtained as statistical estimators.
Our objective is to study SEL theoretically, aiming at establishing convergence results, by simulations, in order to show the increased performance of SEL compared to classical ML.
We also present a practical application whose goal it is to create a prediction model of match results for the women's and men's handball tournaments at the Paris 2024 Olympic Games.