Simplifying Complex Data: How ChatGPT Helps Self-Learners Understand Statistics
Conference
65th ISI World Statistics Congress
Format: IPS Abstract - WSC 2025
Keywords: data science education, interactive learning, llm, statistics education
Session: IPS 699 - ChatGPT: Challenges and Opportunities to Statistical Research and Education
Wednesday 8 October 10:50 a.m. - 12:30 p.m. (Europe/Amsterdam)
Abstract
Using ChatGPT to interpret complex results, such as those from Self-Organizing Maps (SOMs), can be a powerful tool for self-learners tackling intricate data analysis projects. One of the main strengths of this approach lies in ChatGPT's ability to break down and simplify the relationships between the data clusters, allowing users to grasp both macro and micro-level insights effortlessly. I will present two examples. The first is an educational study that was developed for the internal academy of an international organization and deals with geopolitical developments. The second is an application for a large German federal authority.
For instance, in the context of analyzing Africa’s geopolitical alignment with China, Russia, and the West, ChatGPT can visually and verbally dissect the relationship between economic ties and voting patterns in international forums. By explaining SOM-generated clusters based on economic indicators (e.g., debt levels, trade balances), ChatGPT helps users understand how these factors influence a country’s geopolitical leanings. Through this interactive explanation, users can explore key questions like, “How does economic dependency on China affect a country’s stance in international diplomacy?”
This method encourages deeper engagement with data, making it accessible for those who may not have a background in SOMs or machine learning. Furthermore, ChatGPT can generate follow-up questions, guiding self-learners to explore additional layers of complexity, such as the influence of military ties or long-term impacts of debt. Overall, by offering step-by-step clarifications, ChatGPT transforms complex concepts into manageable learning experiences, enabling users to interpret and apply SOM results in real-world contexts.
We are testing this approach in an R&D project with a major German security authority. Pattern recognition methods such as SOMs are used there to better understand risks, identify trends at an early stage and analyze them visually. However, the knowledge gained is not only used by risk analysis experts, but must also be incorporated into the daily work of local authorities. This requires the creation of interactive, automated short reports that can be understood by authority employees without prior knowledge of unsupervised learning methods.
In addition to mere understanding, acceptance also plays a major role. The phenomenon of “functional deviance” is a major problem in the introduction of AI systems. Users bypass the systems, somewhat due to a perceived high level of complexity or a lack of trust. Approaches such as the one we have described could help to promote the compliant use of AI systems by contributing to users' AI literacy. This is highly relevant, not least against the background of Article 4 of the AI Act, which obliges providers and deployers of AI systems to strengthen the AI literacy of users. Due to the possibility of communicating with the system via ChatGPT, the interaction of users with the system can be researched in order to identify complications and vulnerabilities at an early stage.
Figures/Tables
AI-supported_risk-analysis
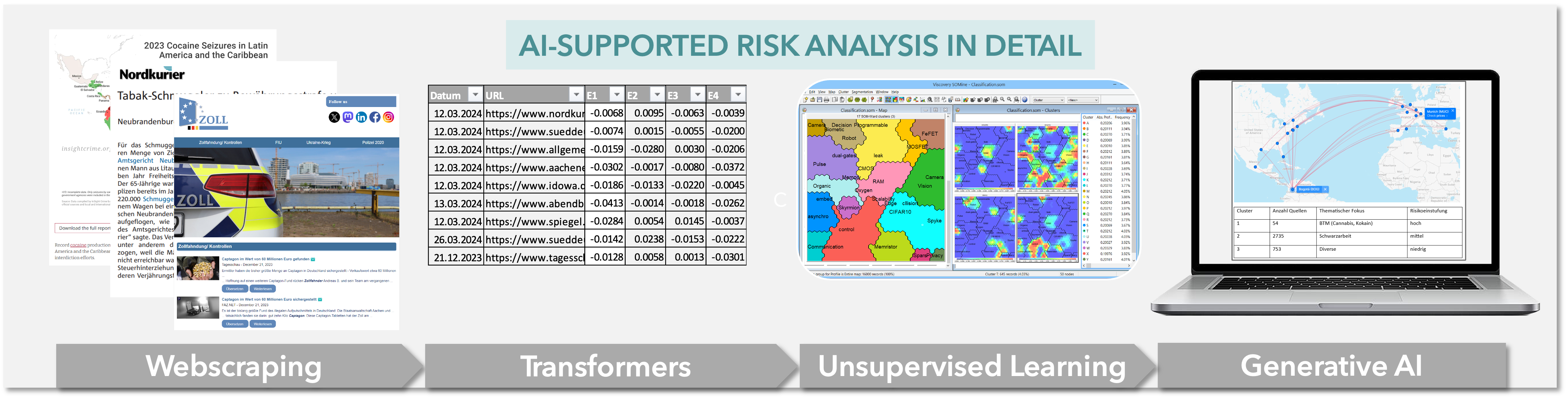