Bayesian Double Generalized Beta Regression for ZIP Code-Level Well-Being Assessment
Conference
65th ISI World Statistics Congress 2025
Format: CPS Abstract - WSC 2025
Keywords: "bayesian, biostatistics, public-health, spatial
Session: CPS 76 - Bayesian Methods for Complex Data Analysis
Monday 6 October 5:10 p.m. - 6:10 p.m. (Europe/Amsterdam)
Abstract
Individual-level assessment of well-being can be used to develop community-level indices that measure wellness and health risks in different geographical regions. These indices typically have bounded support and are updated over time to reflect changes in individual responses or regional characteristics. In this paper, we present a Bayesian double generalized Beta regression framework that utilizes (i) individual-level survey demographic data and ZIP Code-level effects to model the mean of well-being, and (ii) ZIP Code demographic data to model the precision of well-being at the ZIP Code level.
Additionally, our hierarchical prior formulation incorporates spatial and temporal information. ZIP Code neighborhood information is included through a graph Laplacian matrix constructed using driving time between ZIP Code population centroids. We also incorporate a temporal component, wherein posterior estimates of ZIP Code effects from one year inform prior distributions for subsequent years. This allows estimation of ZIP Code effects on well-being using individual data as well as borrowing data from neighboring ZIP Codes and past surveys.
We perform simulations to assess model performance under various spatial patterns and noise settings. The results demonstrate that the model accurately captures the true regression coefficients for all individual and ZIP Code-level demographic variables, as well as the true ZIP Code-level effects. Furthermore, we compare models in which ZIP Code-level posterior effects are used to inform priors in subsequent years versus models in which there is no borrowing of information from one year to the next. This assessment helps determine whether borrowing information temporally yields more accurate posterior estimates in later years. Finally, we apply our model to well-being data from Massachusetts for the years 2021-2023, examining the relationship between different demographic variables and well-being. We highlight which ZIP Codes influence individual well-being in both positive and negative ways.
Additionally, we investigate which ZIP Code-level social determinants of health (SDOH) variables are influencing the ZIP Code-level spatial effects. We conduct a post-hoc analysis by first performing a factor analysis of a set of ZIP Code-level SDOH variables. Then, we use the ZCTA-level spatial effect estimates from the Bayesian Beta regression model as the outcome variable, with the ZIP Code SDOH factors serving as explanatory variables. This analysis allows us to assess which factors play a crucial role in impacting well-being. Please note that we refer to ZIP Code throughout, but ZIP Code Tabulation Area (ZCTA) information is used where appropriate.
This work is an extension of our recent work (paper attached) that proposes a spatially informed statistical model for ZIP code well-being rankings. It uses individual-level demographic predictors and a graph Laplacian matrix to estimate ZIP code effects on well-being. Applied to Massachusetts and Georgia data, it captures demographic patterns and provides community rankings.
Figures/Tables
Simulation ZIP Code effects map
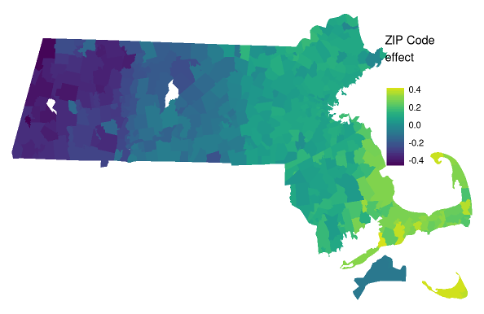
Simulation ZIP Code effects
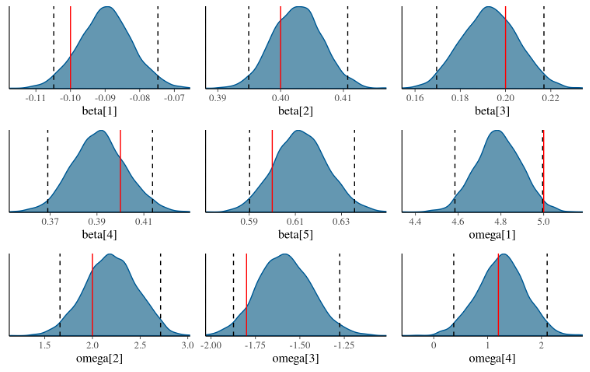
Simulation ZIP Code effect trace plots
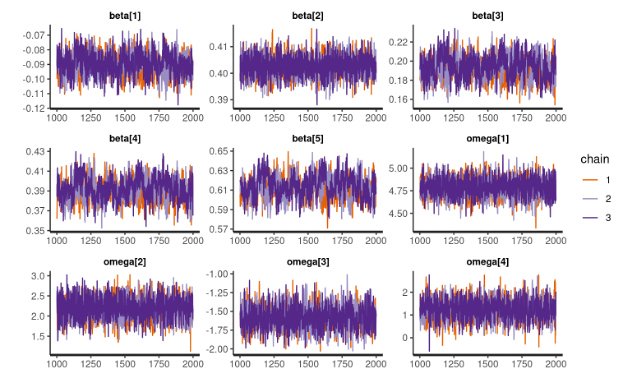