Direction adjusted autoregressive models with applications in psychopathology
Conference
65th ISI World Statistics Congress
Format: CPS Abstract - WSC 2025
Keywords: time_series
Session: CPS 36 - Time Series Analysis and Forecasting
Monday 6 October 4 p.m. - 5 p.m. (Europe/Amsterdam)
Abstract
In the past two decades, the use of experience sampling methodology to collect longitudinal data has taken a steep rise in many areas of psychology and psychiatry, such as studies studying the development of patients with anxiety of depression disorders.
These data typically differ from time series data in other scientific fields in the sense that the number of measurements is low; a time length < 100 is not uncommon. Having few data points restricts the complexity of models that can be applied.
Most approaches in these field therefore rely on the autoregressive AR(1) model, due to the limited number of parameters that need estimation, or small extensions thereof.
Whilst this model has the advantage of being simplistic, it has the drawback of being too simplistic. For many psychopathological processes, an increase over time (e.g. a relapse) can occur much faster than a decrease over time (e.g. a recovery). It is thus not reasonable to assume that the autoregression parameter, denoted inertia in this field, is constant: one can expect a different inertia during periods of increase than during periods of decrease.
In this presentation, I will outline the direction adjusted autoregressive model. This model, derived from the threshold AR model, provides different inertia parameters for each direction of change, whilst keeping the preferable feature of having a small number of parameters. This model is also extended to three states: relapse, recovery and random fluctuation.
Approaches for testing whether the inertia parameter for each state is different are derived. Through simulations I compare the performance of various representations of these model, including that based on differencing and non-parametric bootstrap clustering.
To demonstrate the practical relevance of this model, it is applied to a large-scale dataset from emotion dynamics containing data on 23 emotions from 17 participants. For 262 out of 391 (67%) univariate time series, our model finds statistically different inertia parameters for the two direction-based states. Thus, in many situations this model will provide both a better model fit as well as better insight into the underlying dynamic structure.
Figures/Tables
five
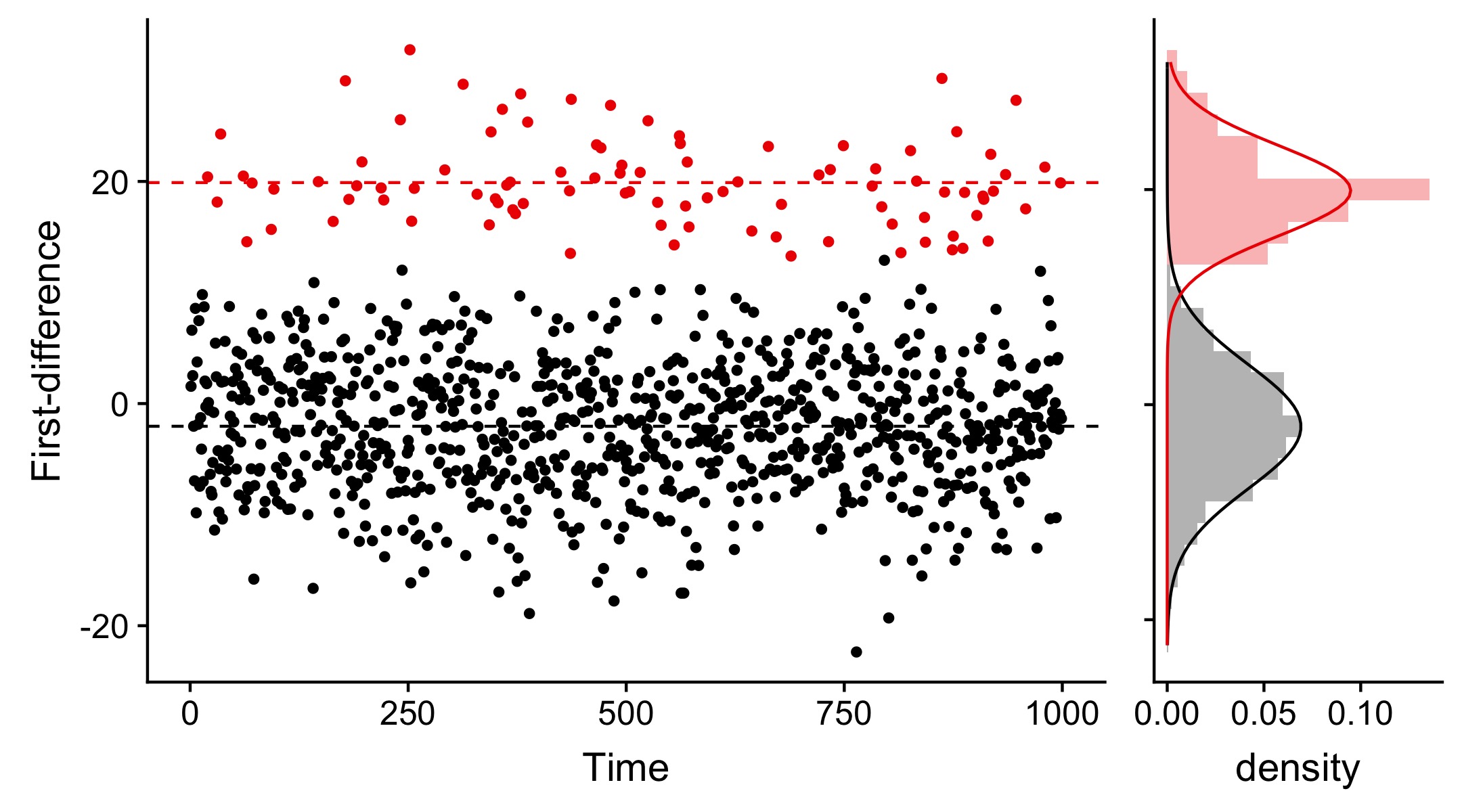