Enhancing Feature Selection Strategies for Imbalanced and High-dimensional Data
Conference
65th ISI World Statistics Congress 2025
Format: CPS Abstract - WSC 2025
Keywords: classification, feature selection, data complexity,
Session: CPS 11 - Dimension Reduction and Clustering Techniques for High-Dimensional Data
Wednesday 8 October 4 p.m. - 5 p.m. (Europe/Amsterdam)
Abstract
Feature selection determines the features that should be included in a model. As data becomes increasingly high-dimensional, feature selection has become a critical topic. An ideal feature selection method should choose the most informative features and eliminate less informative ones, focusing on removing non-informative features to achieve higher accuracy. However, comparing all combinations of features to find the highest accuracy is computationally difficult, time-consuming, and impractical.
Performing feature selection before applying classification predictive models decreases computational time and improves model interpretability. Statistically, estimating fewer parameters is more convenient and reduces the negative impact of non-informative features. Wrapper feature selection methods are among the most commonly used techniques. They evaluate multiple models by adding and/or removing features to find the optimal combination that maximizes overall model performance. Commonly used wrapper methods include Forward Feature Selection, Backward Feature Elimination, and Recursive Feature Elimination (RFE). Typically, wrapper methods rank features by importance as an initial step.
With standard wrapper feature selection techniques, different classification models may select features differently, even for the same dataset. Using synthetic data, we examined four existing feature ordering techniques to identify the most effective and informative ranking mechanism. We propose an improved method to extract the most informative feature subset from the dataset.
Principal Component Analysis (PCA) is a statistical technique used to reduce the dimensionality of high-dimensional datasets. PCA transforms original features into principal components, which are linear combinations of the original features. While principal components are not as interpretable as the original features, we consider the PC loadings and the weights of the features in each linear combination to maintain interpretability. Hence, Our new method uses the sum of absolute values of the first k principal component loadings to order the features, where k is a user-defined, application-specific value. Additionally, it employs a sequential feature selection method to identify the best subset of features. We further compare the performance of the proposed feature selection method with results from the existing Recursive Feature Elimination (RFE) by simulating data for several practical scenarios with a different number of informative features and different imbalance rates. Furthermore, we validated the method using a real-world application on several classification methods. The results based on the accuracy measures indicate that the proposed approach performs better than the existing feature selection methods.
In conclusion, our improved feature selection method effectively identifies the most informative features while minimizing the impact of non-informative ones. Our method enhances model accuracy and interpretability by leveraging PCA loadings and sequential selection. Our comparative analysis and validation demonstrate that this approach is a valuable advancement in feature selection, especially for high-dimensional and imbalanced datasets. This method significantly improves computational efficiency and predictive performance across various applications, including fraud detection, medical diagnosis, and churn prediction.
Figures/Tables
Figure1
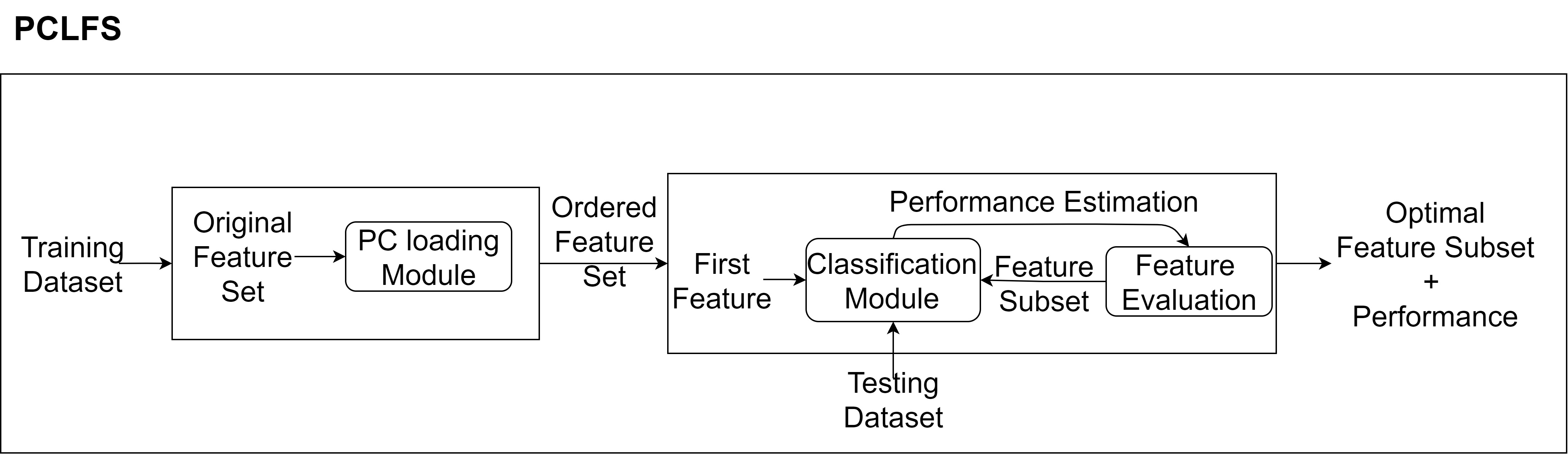
Figure2
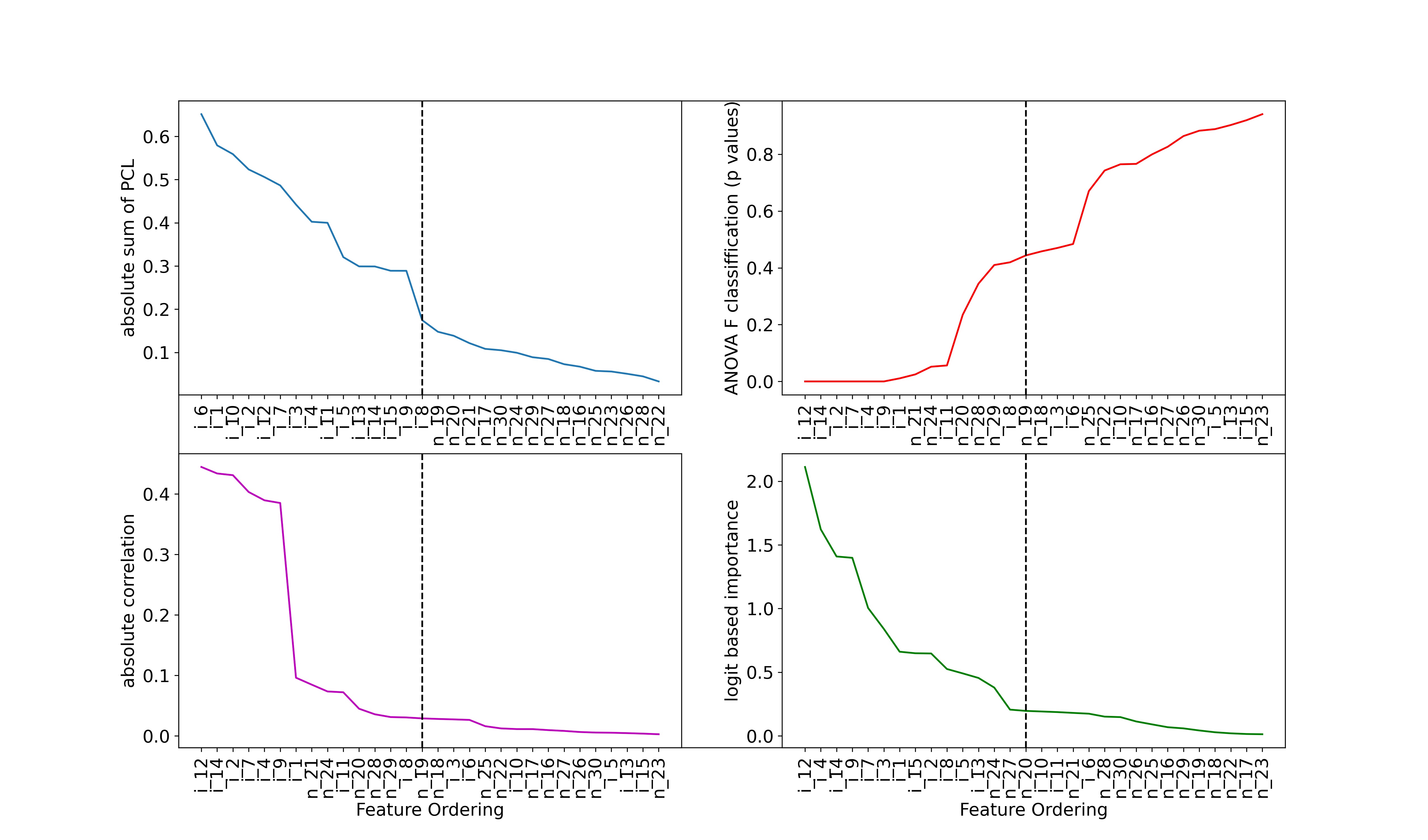
Figure3
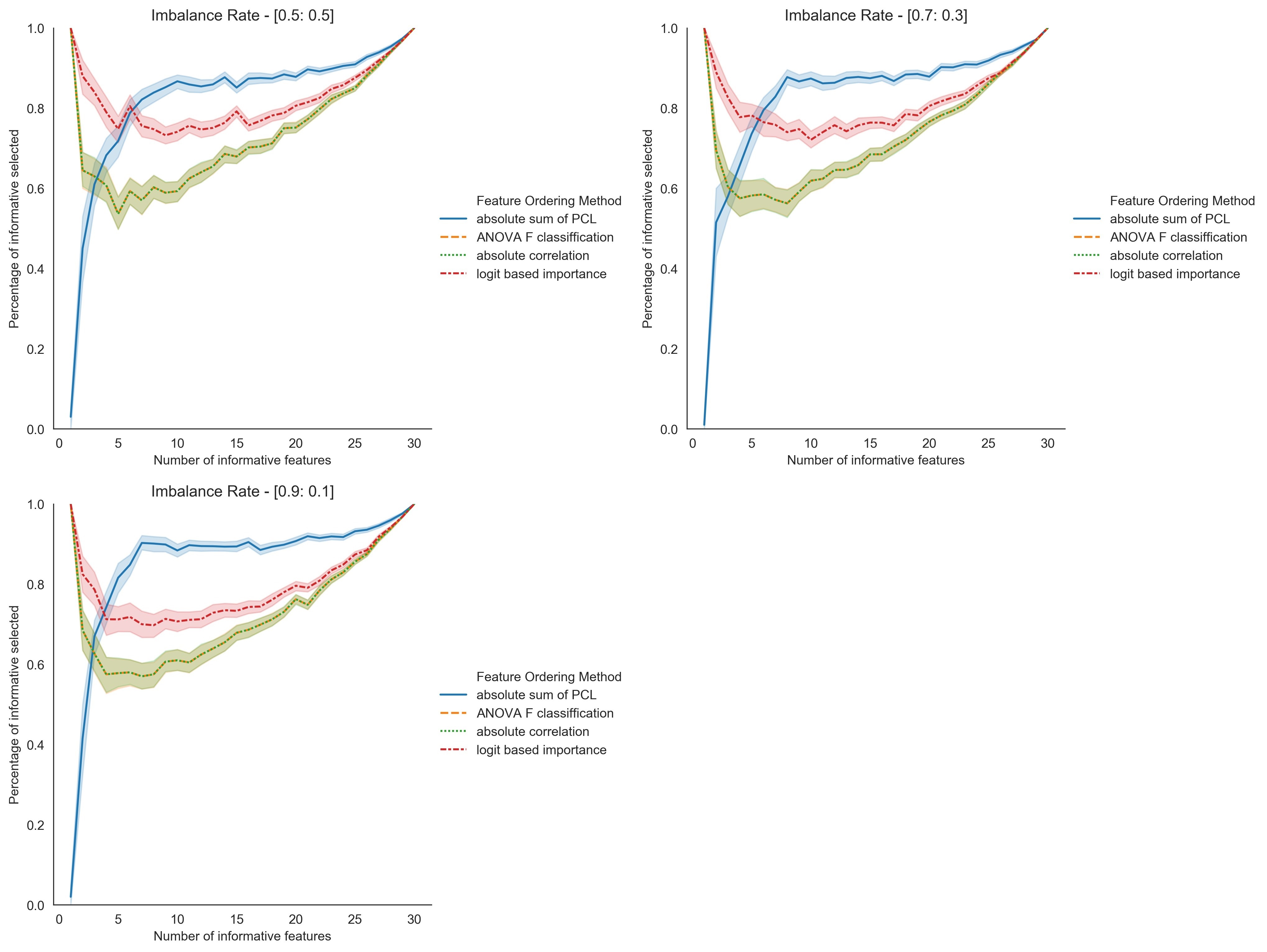
Figure4
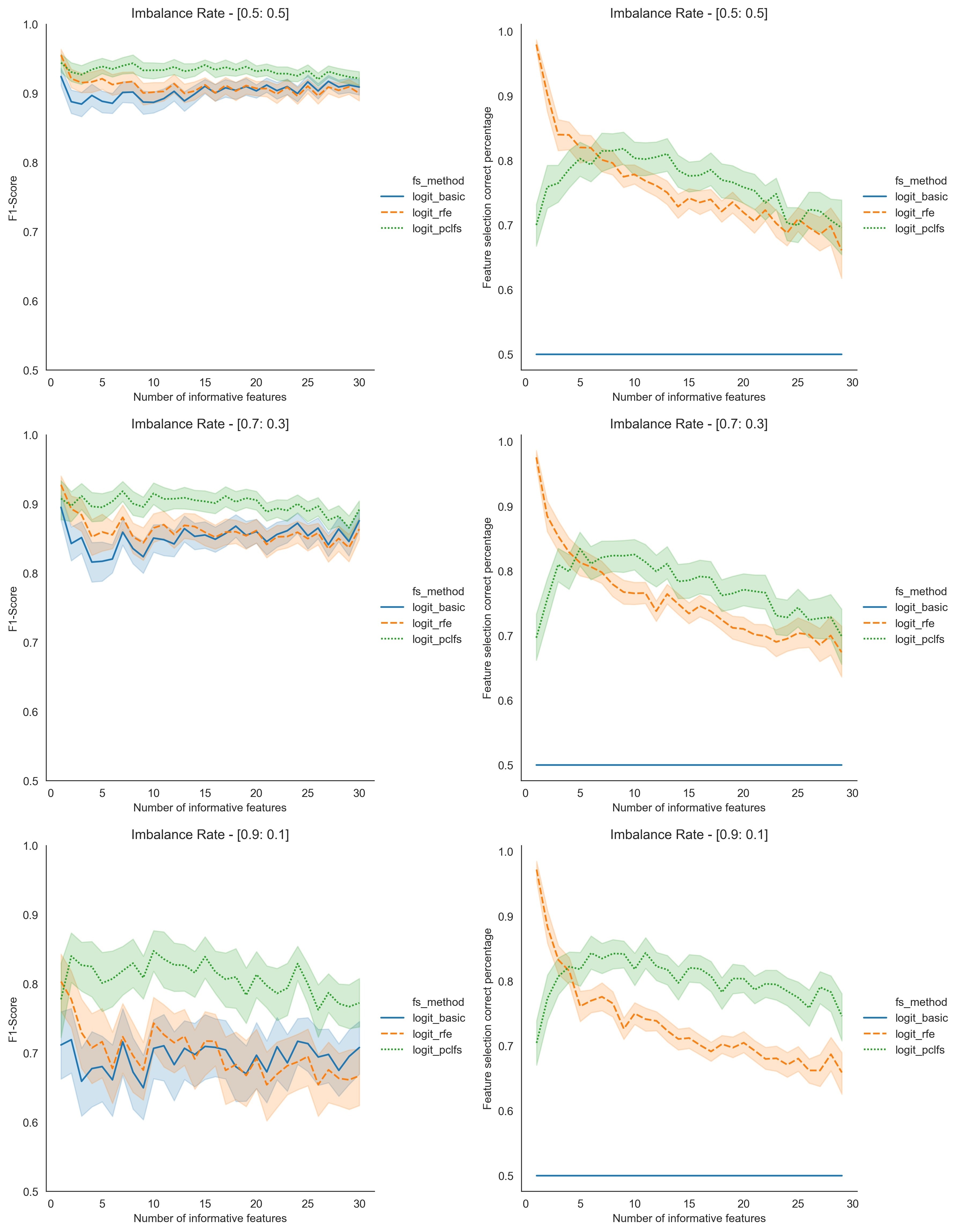
Figure5
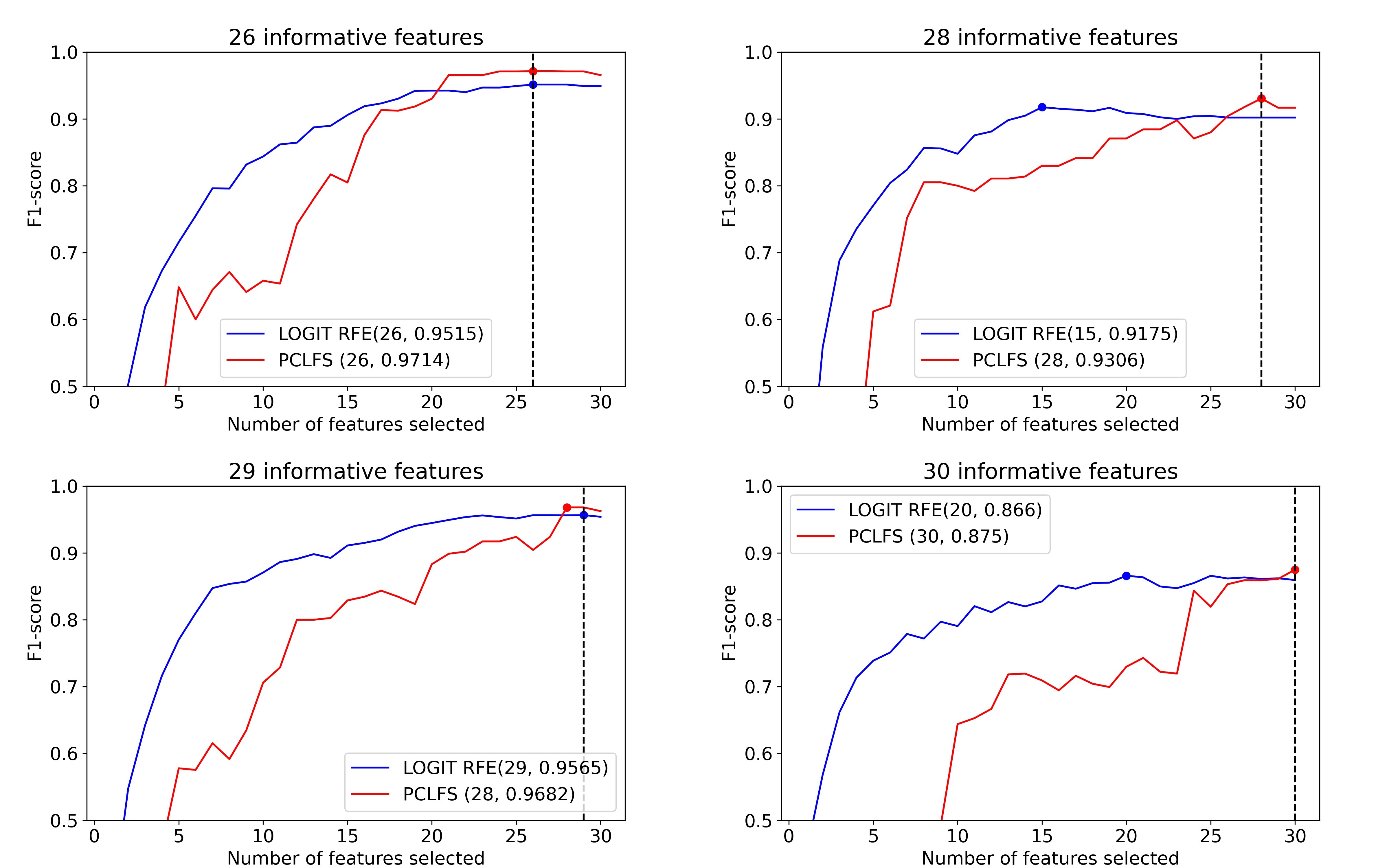