Balancing Predictive Performance and Explainability for Integrating Clinical, Biological, and Radiological Data in the Basque Country COVID-19 Cohort
Conference
65th ISI World Statistics Congress
Format: IPS Abstract - WSC 2025
Keywords: biostatistics, class_imbalance, computerized_clinical_decision_support, covid-19, deep neural networks, deep-learning, interpretability, lasso, logistic model, machine learning, medical-imaging, model_selection, predictive modelling, splines, variable-selection
Session: IPS 950 - Novel Statistical Approaches in Biomarker Discovery, Analysis & Disease Screening
Tuesday 7 October 2 p.m. - 3:40 p.m. (Europe/Amsterdam)
Abstract
AUTHORS
Lander Rodriguez 1, Marta Avalos-Fernandez 2,3, Dylan Russon 2, Irantzu Barrio 4, José M. Quintana-Lopez 5
- 1 Basque Center for Applied Mathematics, BCAM, Spain.
- 2 University of Bordeaux, Bordeaux Population Health Research Center, UMR U1219, INSERM, F-33000, Bordeaux, France
- 3 SISTM team, Inria centre at the University of Bordeaux, F-33405, Talence, France
- 4 University of the Basque Country UPV/EHU, Department of Mathematics, Leioa, Spain; Basque Center for Applied Mathematics, BCAM, Spain
- 5 University of the Basque Country UPV/EHU, Department of Mathematics, Leioa, Spain; Basque Center for Applied Mathematics, BCAM, Spain
INTRODUCTION
In personalized medicine, electronic health records (EHRs) enable predicting clinical outcomes using diverse data such as demographics, clinical histories, biological markers, imaging, treatments, vaccinations, and textual records. Integrating and analyzing these data require sophisticated methodologies, which challenge interpretation. However, interpretability is crucial for gaining trust in healthcare decisions by both professionals and patients.
This study focuses on a retrospective analysis of COVID-19 patients at Galdakao-Usansolo Hospital's emergency departments in the Basque Country from March 2020 to January 2022. The primary objective is accurate mortality prediction while ensuring the integration and interpretability of these diverse data sources. Key research questions include whether integrating radiological and biological data, even for a subset of patients, can optimize prediction models. Additionally, the study explores whether interpretable machine learning methods can compete with deep learning approaches, identifying the most efficient model architectures.
METHODS
This retrospective cohort study included adults (N = 5,504) testing positive for SARS-CoV-2 at the hospital's EDs. Data comprised sociodemographic details, baseline comorbidities, vaccination records, laboratory findings, and mortality status (~10% mortality rate). Additionally, 965 patients had unlabeled frontal chest X-ray images. To integrate data effectively, a categorical variable was created to represent information from X-ray images. Gaussian Mixture Variational Autoencoders with Convolutional Neural Network architecture classified X-ray images into clusters. Patients without X-ray images were categorized under a "missing value" group.
Biological data had varying levels of missingness, with variables missing in >40% of patients excluded and missing values imputed using KNN. The classifier employed was a cost-sensitive Lasso-penalized additive logistic regression, balancing predictive performance with interpretability. Additive functions as 0-degree spline functions simplified variable categorization for clinical interpretation. The Lasso penalty's sparsity combined with stability selection enhanced model robustness. Class weighting considered the empirical class distribution and expert judgment, applied during cross-validation with stratified folds to prevent overoptimistic results.
RESULTS
Figure 1 illustrates variable selection and 0-spline cutoff determination post-stability selection.
CONCLUSIONS
This study demonstrates that integrating radiological and biological data can enhance mortality prediction in COVID-19 patients. It underscores the potential of interpretable machine learning approaches to provide actionable insights for rapid clinical decision-making, thereby improving healthcare delivery efficiency.
REFERENCES
• Avalos M et al. Optimising manual smear review criteria after automated blood count: A machine learning approach. IBICA 2020, Springer, Vol. 1372.
• Dilokthanakul N et al. Deep unsupervised clustering with Gaussian mixture variational autoencoders. ICLR 2017.
• Murti DMP et al. K-Nearest Neighbor based Missing Data Imputation. ICSITech 2019.
Figures/Tables
Figure 1
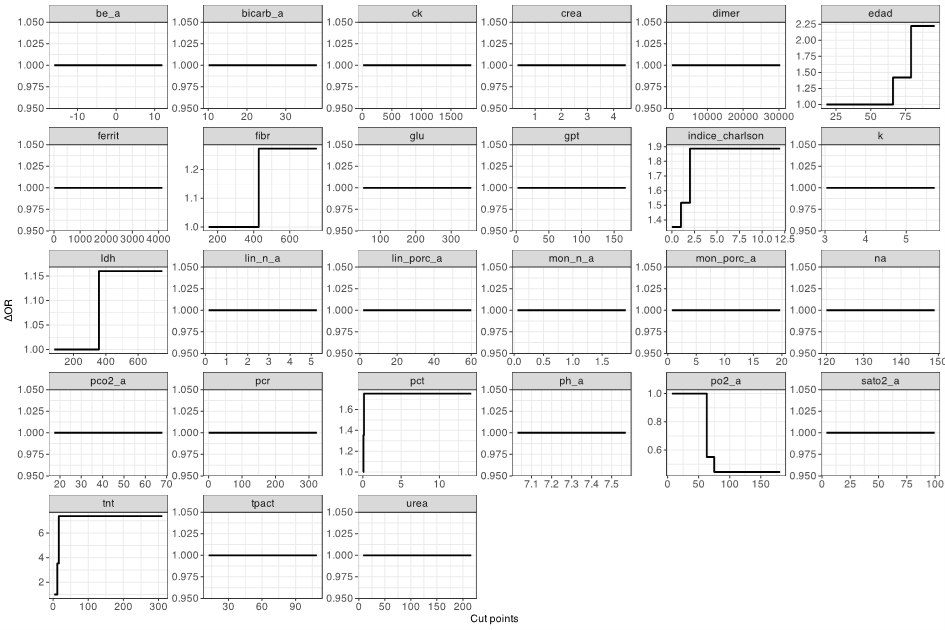