A Hybrid Logistic Regression and Long short term memory Model for Improved Short-term and Ultra Short-term Photovoltaic Power Forecasting
Conference
65th ISI World Statistics Congress 2025
Format: CPS Poster - WSC 2025
Keywords: hybrid, photovoltaic, prediction
Abstract
With the increasing integration of photovoltaic (PV) systems into the energy grid, there is a growing need for improved methods to predict the performance and reliability of PV systems. Current research shows that hybrid models are superior to single models. This paper introduces a new hybrid model for PV power prediction. Since solar radiation is the most important factor affecting PV power output, the relationship between different kinds of radiation and power is first analyzed. Power is then classified using logical classification, where the genetic algorithm helps to select the parameters automatically. The long short term memory (LSTM) model can automatically select which unimportant information to forget, effectively dealing with the problem of discontinuous time intervals.
Combining the optimized LSTM model with the logistic regression allows for ultra-short-term and short-term predictions using both seasonal data and any consecutive 90-day data. By comparing three models—our proposed model, the LSTM model, and the persistence model—we can conclude that the results from the LSTM model with logistic regression meet the requirements of ASHRAE Guideline 14, which is one of the major guidelines for PV systems. Moreover, the RMSE value can be controlled within 1. The three different error evaluation metrics—RMSE, CV, and MBE—validate the superiority of our proposed model in terms of accuracy, stability, and consistency across various aspects.Different installed capacities of various PV plants do not affect the model's predictions. Data from five PV plants located in China's Jiangsu Province in 2023 are used to validate the model's effectiveness and reliability.
Figures/Tables
Comprehensive Overview of the Overall Process and Methodologyof the Proposed Model
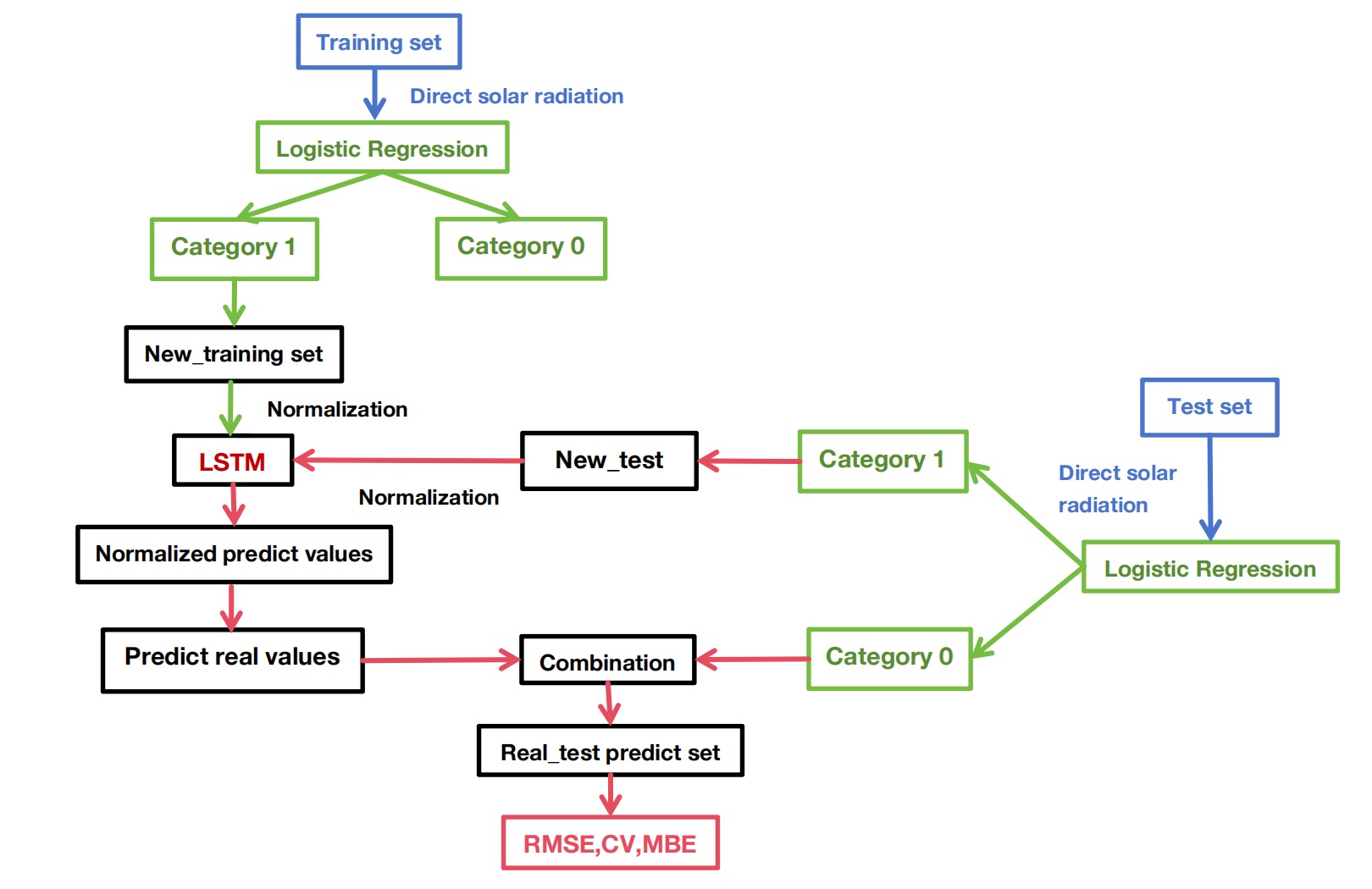