Enhanced Land Cover Classification Using Deep Learning with High-Resolution Freely Available Satellite Imagery
Conference
65th ISI World Statistics Congress
Format: CPS Abstract - WSC 2025
Keywords: deep-learning, land-cover-classification, sattelite-data
Session: CPS 23 - Statistical Methods for Environmental and Climate Data Analysis
Tuesday 7 October 4 p.m. - 5 p.m. (Europe/Amsterdam)
Abstract
Title: Enhanced Land Cover Classification Using Deep Learning with High-Resolution Freely Available Satellite Imagery
Satellite imagery, especially from the Sentinel-2 mission, has become a cornerstone in various applications ranging from environmental monitoring to land cover classification. Sentinel-2, with its multispectral capabilities and frequent revisits (5 days), offers various information for earth observation. Advantages and features of Sentinel-2 imagery made it a preferred choice for this study.
This study presents the development and implementation of a deep learning model for land cover classification using freely available Sentinel-2 and Landsat 8 satellite imagery from the Sentinel Hub.
The primary objective was to develop an accurate deep learning model for enhanced land cover classification by leveraging the complementary strengths of both satellite datasets and compute the area occupied by each of the land covers in Rwanda. This was aiming at providing accurate and timely data and statistics as currently the land cover classification in Rwanda is updated once in three years.
Images were downloaded from the Sentinel Hub using existing ground truth data locations by leveraging data science techniques and underwent a preprocessing phase that included cloud cover and shadow removal, labelling, Data normalization, Augmentation and splitting for the model training. For clouds and shadow removal, cloudy pixels in Sentinel-2 images were replaced with corresponding pixels from Landsat 8 images which were cloud-free, ensuring cleaner and more reliable input data. Additionally, Sentinel-2 Scene Classification Layer (SCL) images were employed to accurately identify and delineate areas covered by water. The preprocessed imagery was then utilized to train a deep learning model by leveraging pre-trained models such as Unet, Unet++, Linknet and Deeplabv3 and then evaluated using different metrics such as Intersection over Union (IoU), Dice score coefficient, and pixel accuracy on the test set to assess the model’s performance and the Unet++ demonstrated robust performance in classifying various land cover types. The model was then used to classify land cover in each of the district of Rwanda and an AI Web powered Application was developed to create a user friendly interface to enable those without coding skills to get the classification results by themselves.
The results indicate that integrating data from multiple satellite sources and employing advanced preprocessing techniques can significantly improve land cover classification accuracy and timely data insights for decision making. This approach holds promise for applications in environmental monitoring, urban planning, and natural resource management.
Figures/Tables
workflow of the project
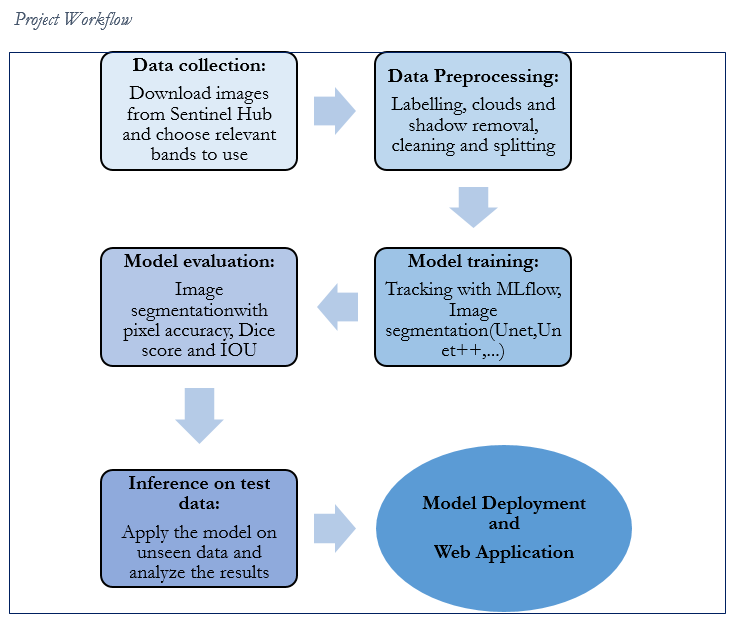