Optimizing GRDP Predictions: Evaluating Temporal Disaggregation Techniques with Multisource Data Integration
Conference
65th ISI World Statistics Congress
Format: CPS Abstract - WSC 2025
Keywords: disaggregation, temporal
Session: CPS 83 - Predictive Analytics and Nowcasting
Monday 6 October 5:10 p.m. - 6:10 p.m. (Europe/Amsterdam)
Abstract
The increasing demand for detailed economic data, such as Gross Regional Domestic Product (GRDP), reflects local governments' need for comprehensive economic indicators. However, providing high-frequency economic indicator series for smaller economies faces challenges due to limited data availability at lower regional levels. While big data could assist, its access and utilization present constraints. Temporal disaggregation offers a potential solution by converting low-frequency data into high-frequency series, but its implementation requires careful scrutiny. This study explores the reliability of the temporal disaggregation technique for GRDP, identifies factors determining the quality of predicted disaggregated series, and assesses the potential role of big data. The empirical strategy involves evaluating the accuracy of quarterly high-frequency series generated through temporal disaggregation applied to annual GRDP data. The simulation focuses on Sumatera Barat province's agricultural and accommodation sectors. Existing quarterly GRDP series are compared against predicted values using Mean Absolute Percentage Error (MAPE) and the Spearman correlation coefficient to assess alignment in quarterly sign change movements. Temporal disaggregation is performed twice: first without additional indicators and then with them. For the agricultural sector, the additional indicator is the quarterly series of rice production. For the accommodation sector, Google Trend indices (keyword: “Hotel”) and the number of tourist arrivals at the local airport serve as additional indicators. This study also attempts to disaggregate GRDP into high-frequency series across different levels for the agricultural sector: the parent sector (Agriculture, Fishing, and Forestry), subsector (Agriculture), and sub-subsector (Food Crops). The findings indicate that disaggregation without additional indicators leads to oversmoothing of the high-frequency series. Indicators that account for a higher share in the target variable tend to result in more accurate predicted series. The study highlights the promising potential of utilizing easily accessible big data indicators in GRDP disaggregation, suggesting that carefully selected additional indicators can significantly enhance the quality of high-frequency economic data derived from low-frequency series.
Figures/Tables
Rplot01
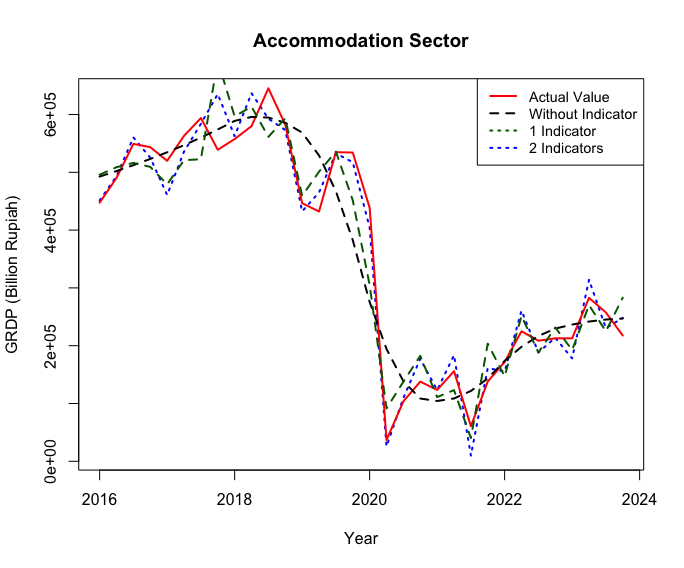
Rplot
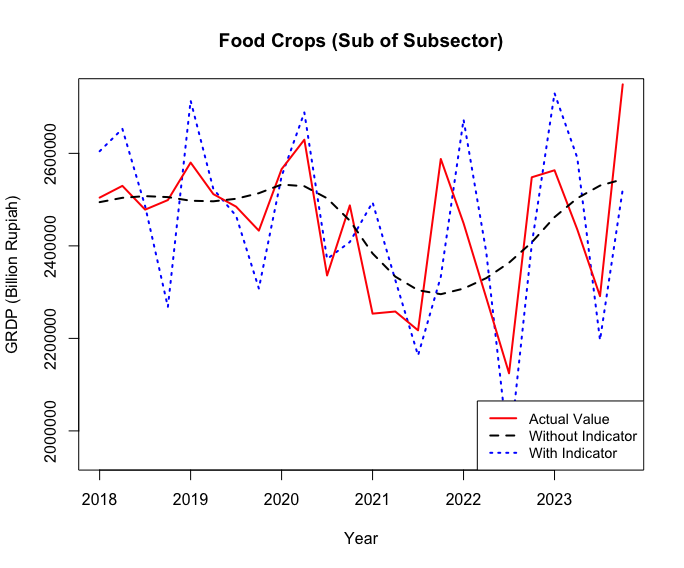