Research on Optimizing Seasonal Adjustment of Time Series Data Using DTW and Machine Learning
Conference
65th ISI World Statistics Congress
Format: CPS Abstract - WSC 2025
Keywords: dtw, machine learning, random-forest, randomforest, seasonal_adjustment, x-13_arima_seats
Session: CPS 49 - Seasonal Adjustment and Time Series Analysis for Official Statistics
Monday 6 October 5:10 p.m. - 6:10 p.m. (Europe/Amsterdam)
Abstract
This study introduces a methodology aimed at enhancing and streamlining the process of seasonal adjustment with regards to time series data within the economic domain. The significance of seasonal adjustments is to reveal fundamental trends and cycles by removing seasonal fluctuations from time series data from national statistics.
However, the seasonal adjustment process becomes more complex due to economic fluctuations, requiring practical experience and industry-specific knowledge. This study focuses on the question, "Can practical experience optimize seasonal adjustments?" and aims to identify this issue and propose a solution.
The study focuses on the "Mining Manufacturing Industry Trend Survey" by the Statistics Korea. It analyzes the monthly production index (both raw and seasonally adjusted) for manufacturing sectors with distinct seasonality. Using Python to automate the X-13 ARIMA SEATS program generated diverse option combinations, allowing verification of similarity between seasonally adjusted and forecast indexes via DTW. A random forest regression model identified an optimal model with high explanatory power (R² scores are 0.93 to 0.99). Key features include Sum Seas MA for the C265 industry and D11F 3yr for the C331 industry. Industry-specific application of these elements is expected to enhance seasonal adjustment efficiency.
This study has enabled the automation of repetitive tasks to lessen workload, with the incorporation of DTW and machine learning enabling the identification of pertinent option combinations and diagnostic outcomes tailored to distinct industry classifications. Furthermore, the results of this research study are anticipated to improve the operational efficiency of national statistical bodies, thereby supporting more accurate economic assessments and informed policy-making processes.
Figures/Tables
Figure 1 Comparison of Traditional Process and Python Automation Process for X-13A-S
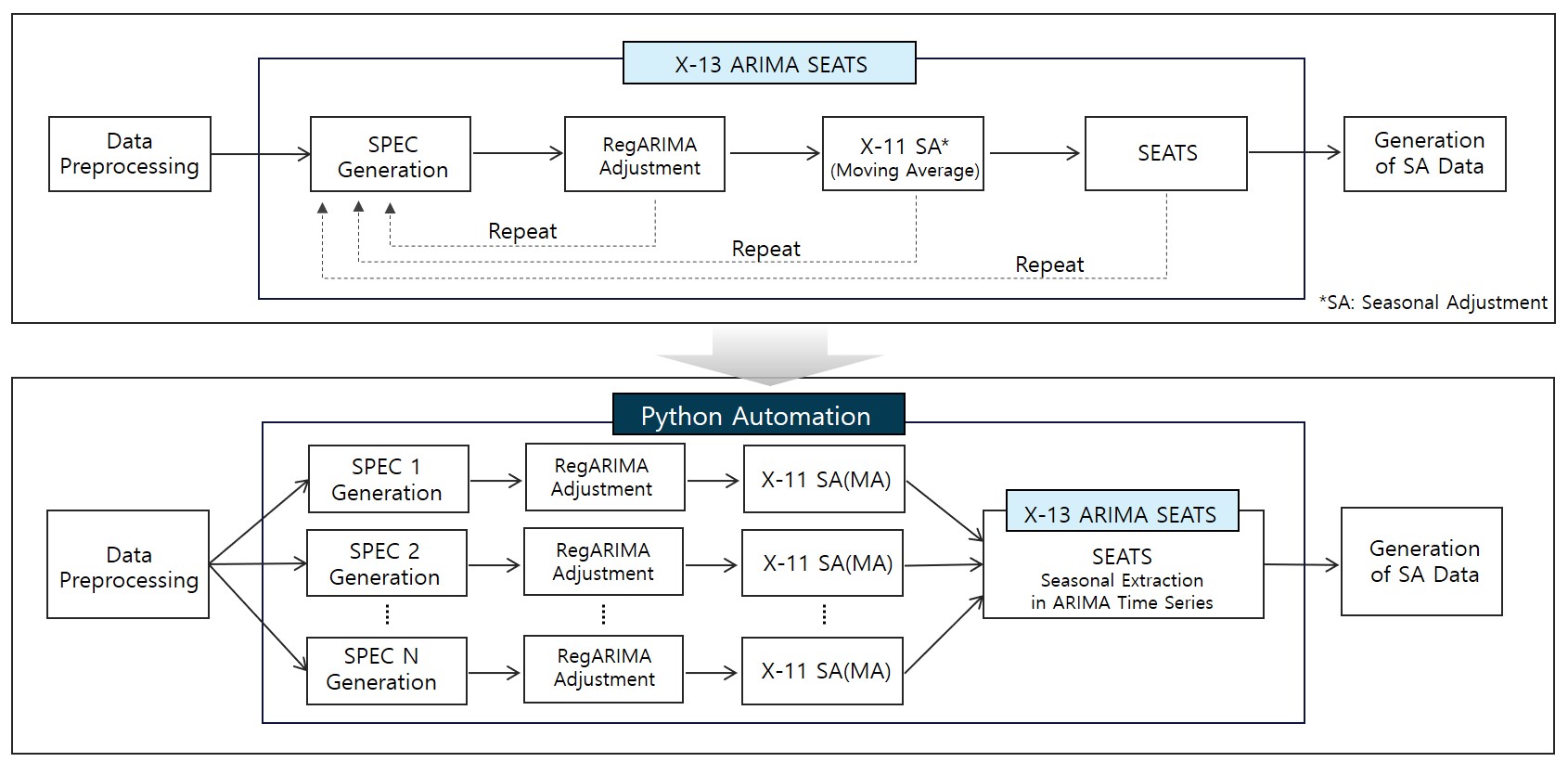
Figure 2 C291(the industry of general purpose machinery manufacturing) Production Index
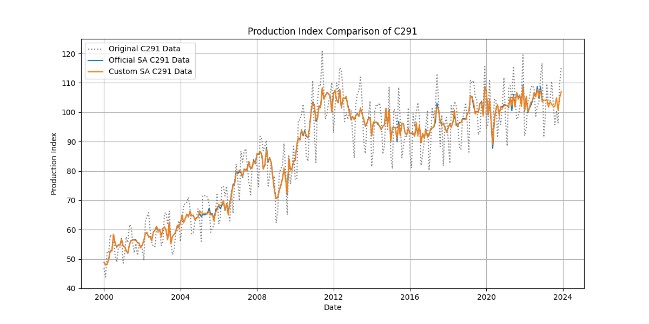
Figure 3 C152(the sector of footwear and shoe parts manufacturing) Production Index
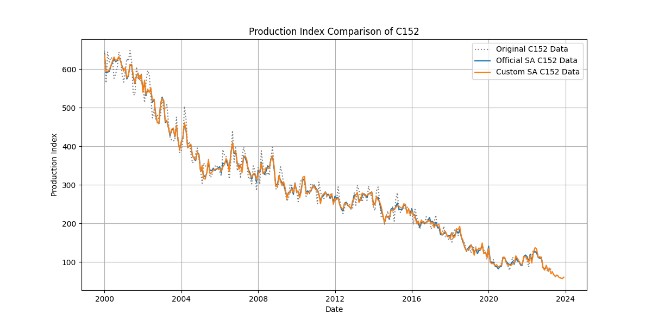
Figure 4 Feature Importance of C265(manufacturing of imaging and audio equipment)
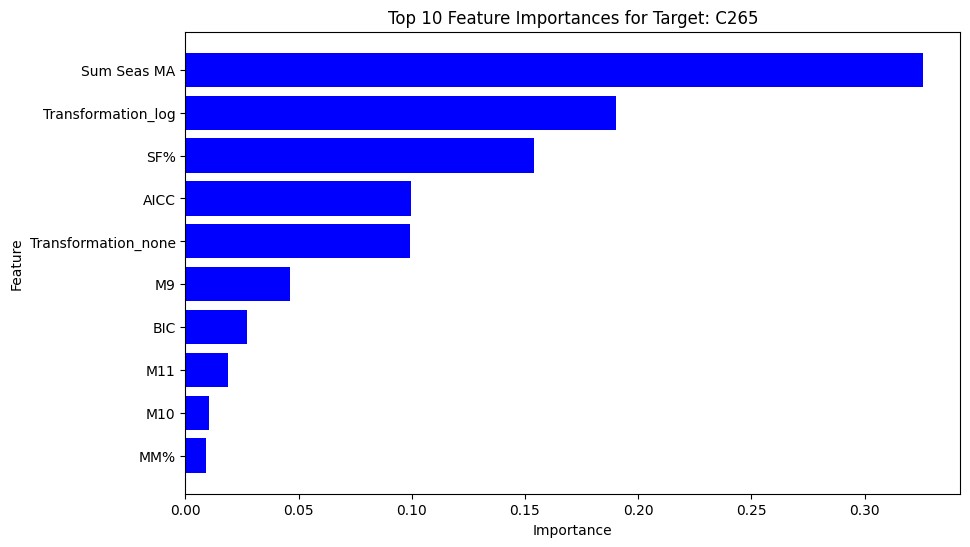
Figure 5 Feature Importance of C331(manufacturing of precious metals and jewelry)
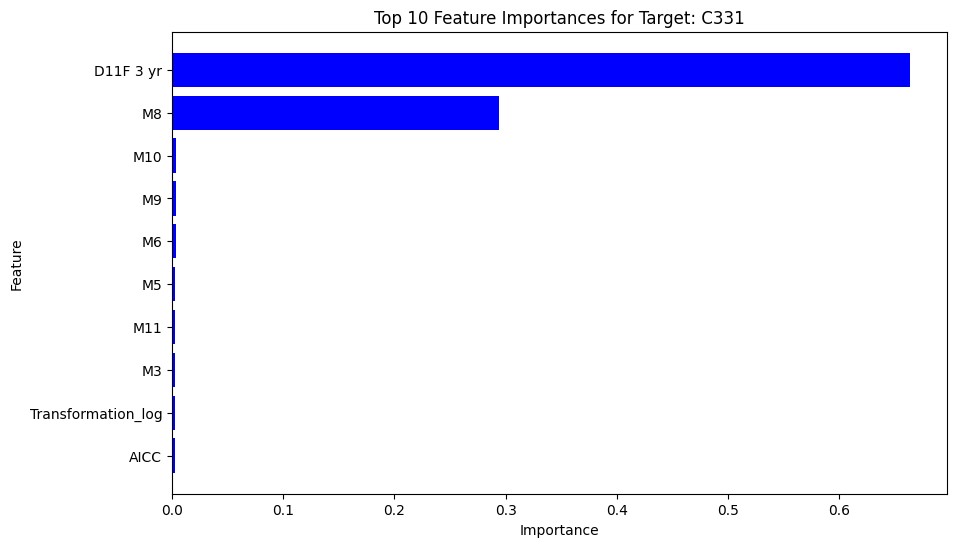