Bayesian Analysis of Flexible Heckman -Selection Models Using Hamiltonian Monte Carlo
Conference
65th ISI World Statistics Congress 2025
Format: CPS Abstract - WSC 2025
Keywords: bayesian modeling, bayesian_model, statistics
Session: CPS 76 - Bayesian Methods for Complex Data Analysis
Monday 6 October 5:10 p.m. - 6:10 p.m. (Europe/Amsterdam)
Abstract
The Heckman selection model is widely employed in econometric analysis, as well as in other social sciences, to address sample selection issues in data modeling. Its fundamental assumption hinges on the normality of error terms in both the outcome and the sample selection equations. Extensions to this model have been pursued due to issues of skewness and high kurtosis in real-world applications. Among these extensions, the first proposal was the selection t-model, where random errors follow a bivariate Student-t distribution. Another more recent notable extensions are the Heckman selection skew-normal and contaminated normal models, in which the error terms follow bivariate contaminated-normal and skew-normal distributions, respectively. Parameter estimation in these models has been done by using likelihood-based methods, such as the EM-algorithm implemented in the R package HeckmanEM. In this work, we explore these selection models using a Bayesian approach facilitated by the Stan software. Our objective is to compare the performance of the normal, Student’s-t, contaminated normal and skew-normal selection models in real-world applications, aiming to address the complexities introduced by non-standard error distributions. We also conduct several simulation studies to evaluate the performances of the four types of models.
Figures/Tables
Estimatesmodeldatset
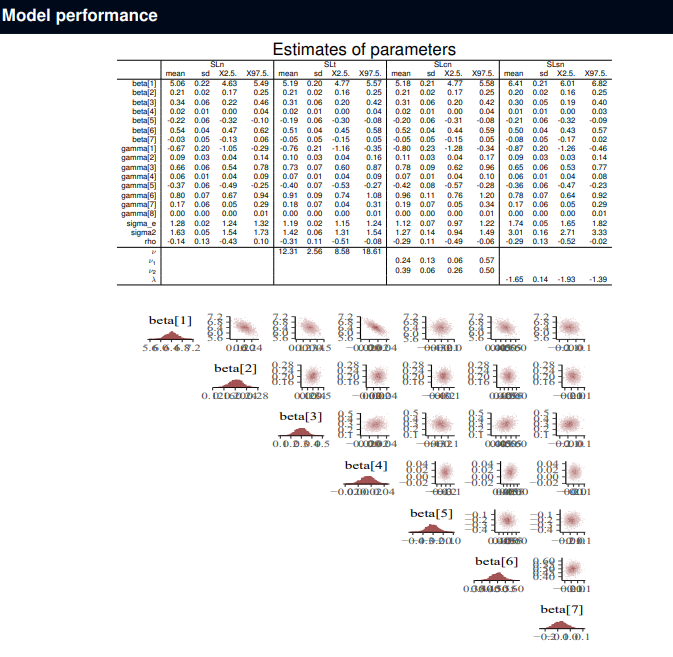