Stochastic Volatility in Mean: Efficient Analysis by a Generalized Mixture Sampler
Conference
65th ISI World Statistics Congress
Format: CPS Abstract - WSC 2025
Keywords: leverage effect, markov chain monte carlo, stochastic volatility
Session: CPS 12 - Financial Modelling and Volatility
Tuesday 7 October 5:10 p.m. - 6:10 p.m. (Europe/Amsterdam)
Abstract
This study presents a new Bayesian approach for analyzing stochastic volatility in mean (SVM) models using a generalized mixture sampler. Our method approximates the non-central chi-squared distribution as a mixture of normal distributions, which makes the sampling process much more efficient. This innovation helps to handle large and complex datasets more easily, addressing common computational challenges.
We start by explaining the theoretical framework of our generalized mixture sampler, showing how it simplifies the sampling process. By using the properties of the normal mixture, we reduce the computational burden of traditional methods and make the sampling from posterior distribution more efficient. This reduction in computational complexity and high efficiency allows our method to be applied to more extensive datasets and more intricate models without significant increases in processing time.
We then extend this method to include leverage effects, which are important for capturing the asymmetry often seen in financial time series data. Including leverage effects makes our model better at reflecting the real behavior of financial markets, where negative and positive shocks can have different impacts on volatility. This extension is crucial for accurate modeling of financial time series, as it captures the nuances of market movements more effectively.
To test our method, we apply the SVM model with and without leverage to both simulated and real-world data. Our simulation study shows that our method can achieve efficient parameter estimates, with less autocorrelated MH sampling and less computational time compared to traditional methods. This demonstrates the practical benefits of our approach in a controlled environment.
In the real-world application, we focus on excess holding yields, an important measure in financial econometrics. By applying our SVM model with leverage to this dataset, we show how it effectively captures the complex movements of financial returns. The results indicate that our model provides reliable estimates and fits the data well, proving to be more efficient than existing models. This empirical validation highlights the practical applicability of our method in analyzing real financial data.
In conclusion, our generalized mixture sampler is a significant advancement in the Bayesian analysis of SVM models. By offering a more efficient way to estimate parameters and volatility, it makes it easier to apply SVM models to large and complex datasets. This is valuable for financial econometrics, allowing for better analysis of market dynamics. Future research might explore applying this method to multivariate volatility models and high-frequency financial data, potentially extending the benefits of our approach to a broader range of applications in finance and beyond.
Figures/Tables
True and approximation densities
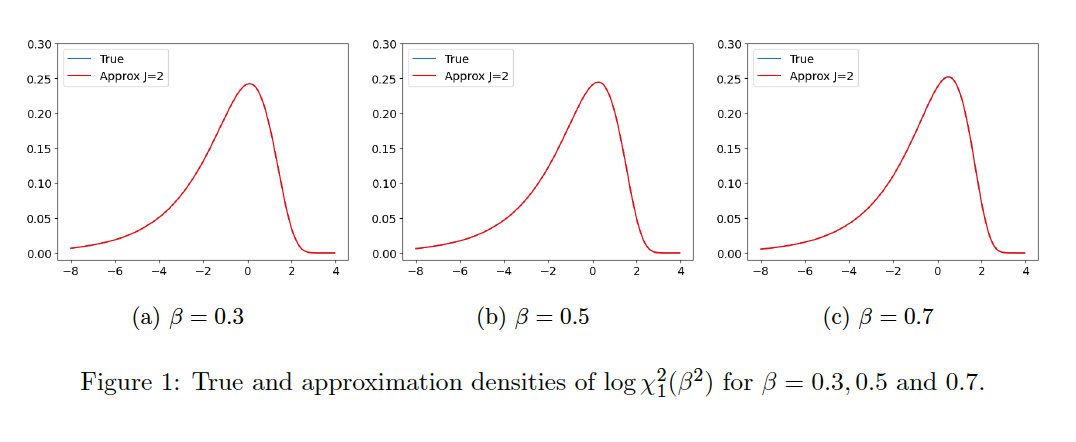
Differrence of the two densities
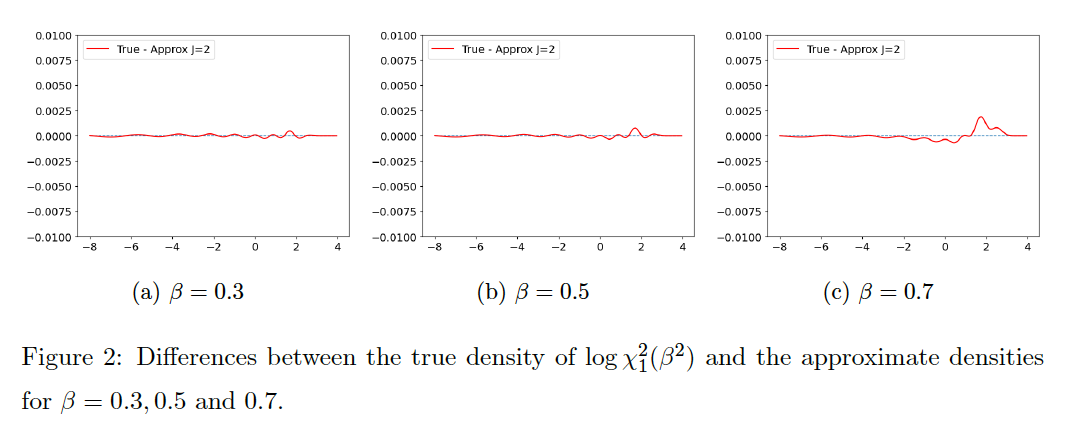
Simulation result
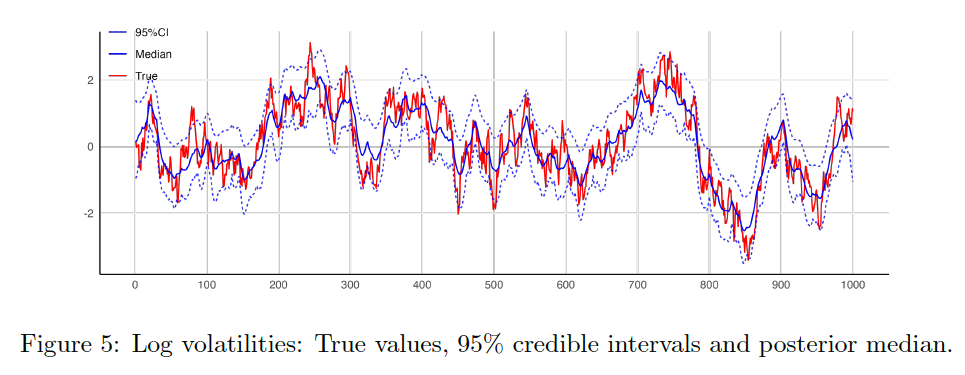
Summary of simulation study
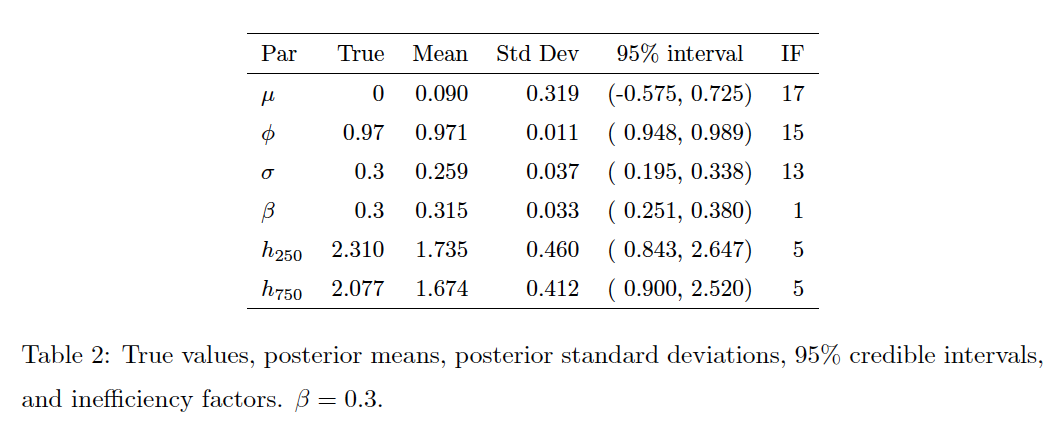