Application of Large Language Models for Dissemination of Statistics
Conference
65th ISI World Statistics Congress
Format: CPS Poster - WSC 2025
Keywords: artificial-intelligence, data, official statistics
Abstract
The evolution of large language modes(LLMs) has indeed been shaped by decades of research in natural language processing(NLP) since the 1940s after World War II, however, the advent of the transformer architecture by google in 2017 created a major breakthrough paving the way for the development of large language models like bidirectional encoder representations from transformers(BERT),generative pre-trained transformer(GPT), and T5. These models have demonstrated unprecedented capabilities in understanding and generating human-like text, significantly advancing tasks such as text summarization, translation, and question answering.
Presently, large pre-trained language models have been shown to store factual knowledge in their parameters especially for downstream tasks, something that domain specific disciplines like medicine, finance and statistics can leverage on. In this study, retrieval-augmented generation(RAG) which combines pre-trained parametric and non-parametric memory generation has been explored for question and answer tasks involving official statistics. This provides a foundation of applying generative technology in official statistics, offering a new avenue for data accessibility and understanding
Moreover, the study explored the challenges and opportunities associated with integrating generative technologies within national statistics offices (NSOs), aiming to facilitate their adoption while carefully considering the potential effects on the fundamental principles of official statistics.
Figures/Tables
RAG pipeline
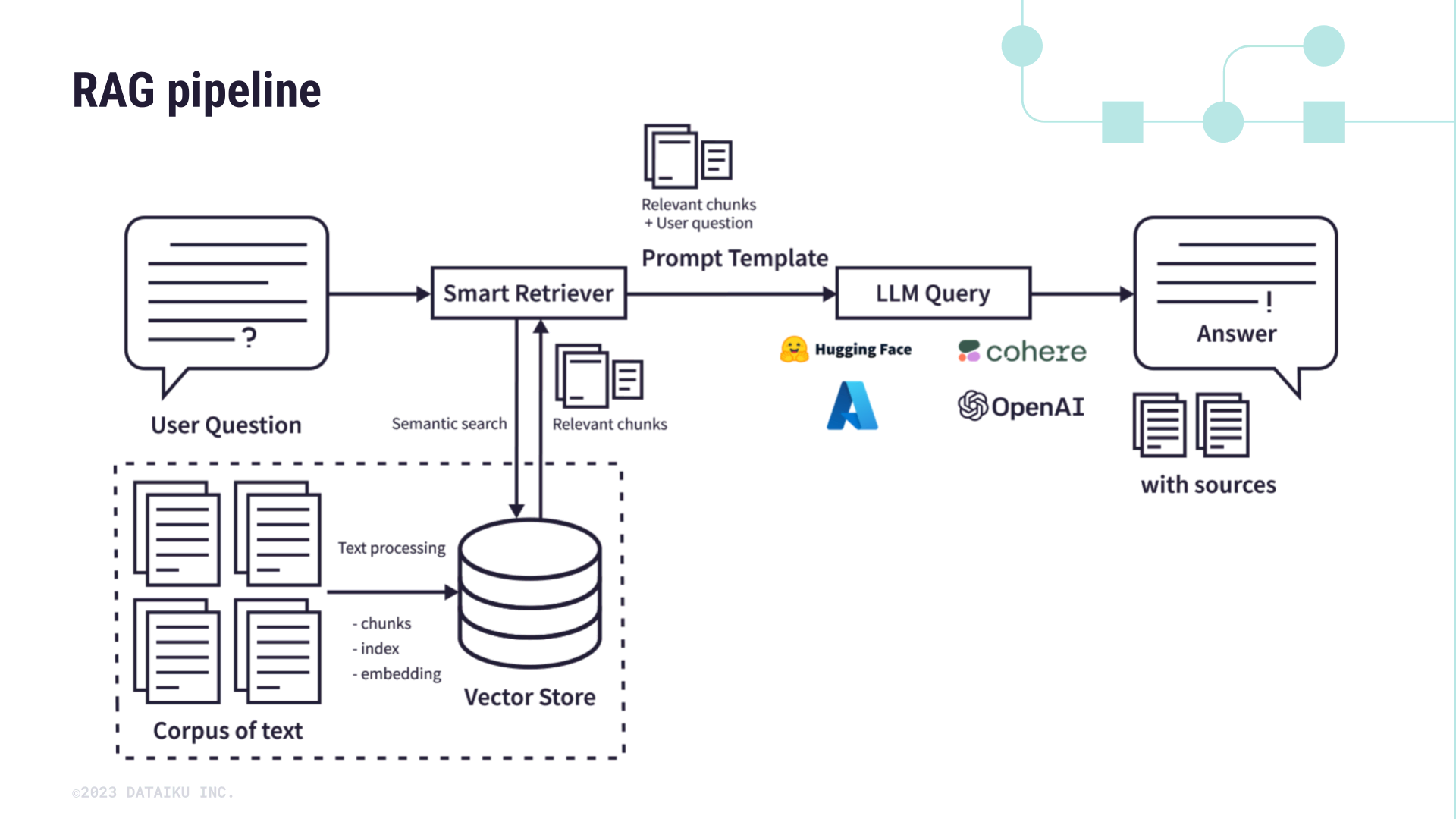
system process 2
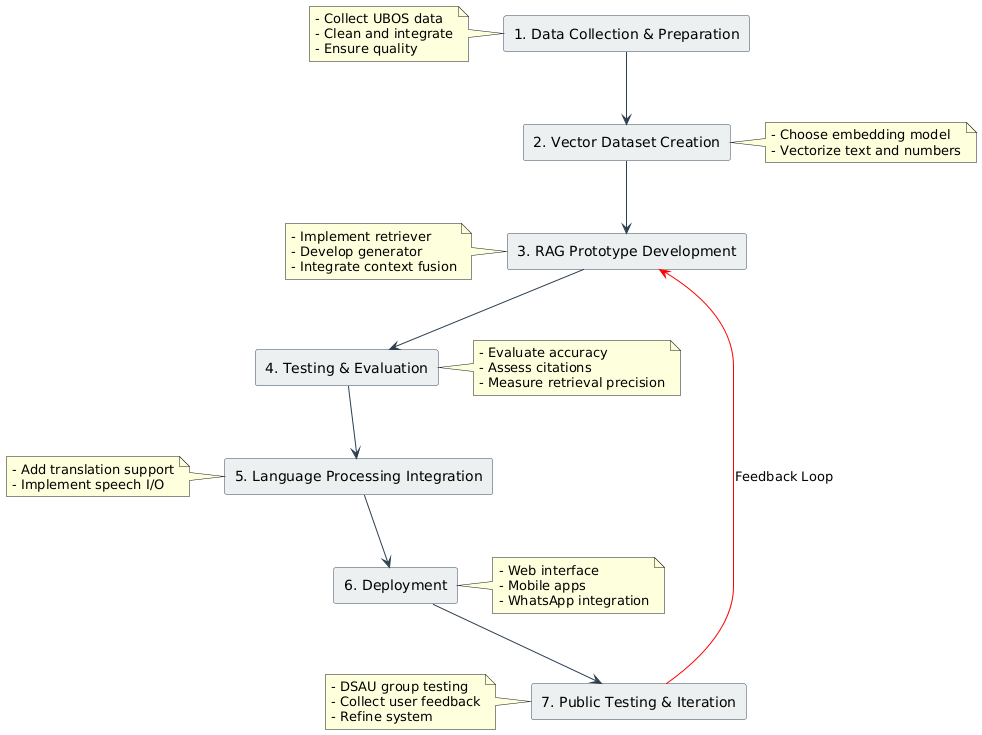
system process
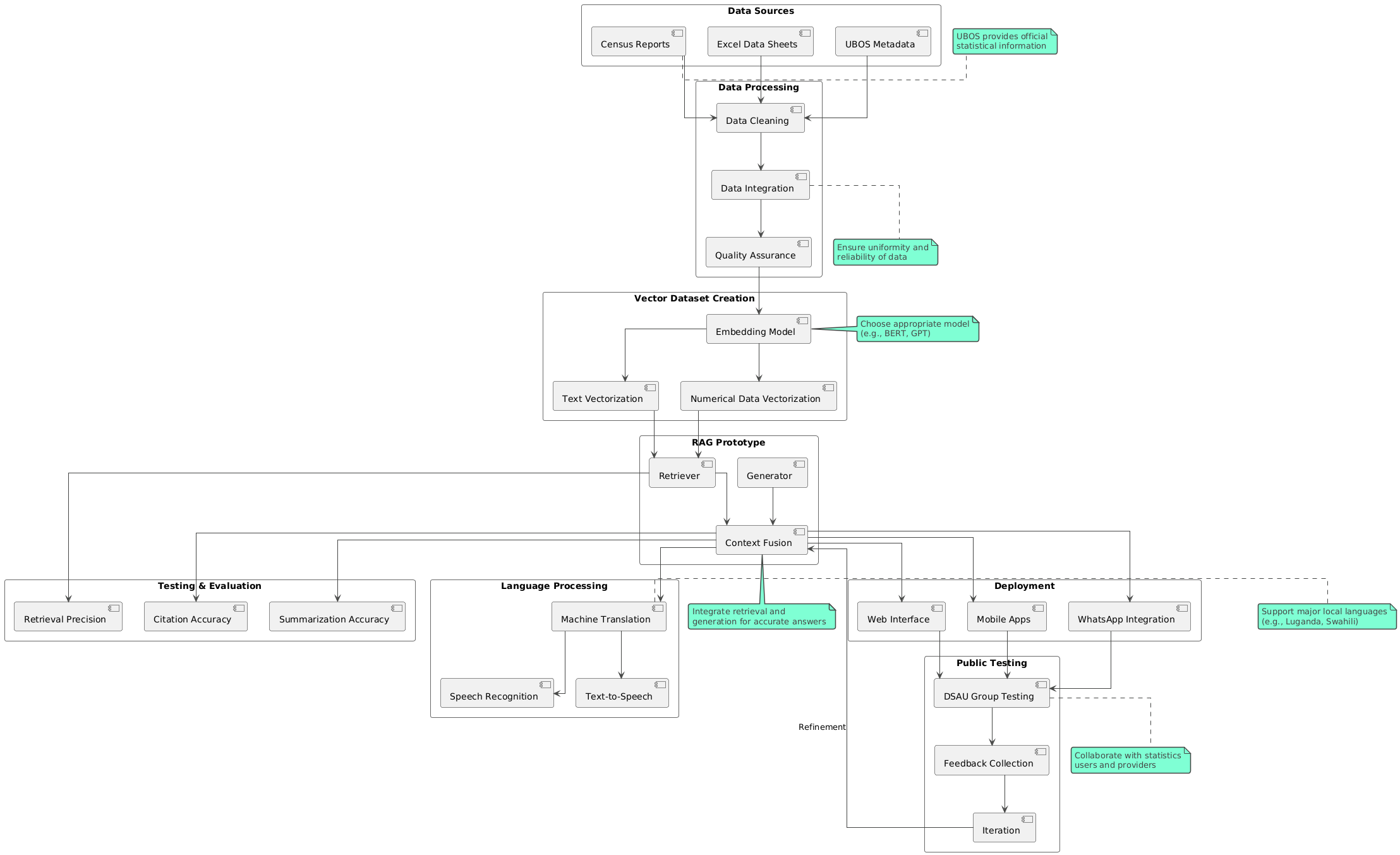