Mixture-Based Approximately Unimodal Likelihood Model for Ordinal Data
Conference
65th ISI World Statistics Congress
Format: CPS Abstract - WSC 2025
Keywords: ordinal_data, ordinal_regression, unimodal
Session: CPS 14 - Ordinal Data and Tree-Based Methods
Wednesday 8 October 4 p.m. - 5 p.m. (Europe/Amsterdam)
Abstract
Ordinal regression (OR, also called ordinal classification) is the classification of ordinal data, in which the underlying target variable is categorical and considered to have a natural ordinal relation for the underlying explanatory variable. Various practical tasks have been tackled within the OR framework such as face-age estimation, credit or movie rating, and analysis of questionnaire survey. Yamasaki (2022, TMLR) supposed that the conditional probability distribution of the target variable given a value of the explanatory variable tends to be unimodal as a characterization of the natural ordinal relation of ordinal data, and verified that this hypothesis holds well for real-world data that previous OR studies have treated as ordinal data. Also, he developed unimodal likelihood models with different levels of representation ability that guarantee the predicted conditional probability distribution is unimodal, and showed that those models perform well in prediction of the conditional probability and OR tasks for real-world ordinal data: the larger the number of available training data, the more suitable the model was with higher representation ability. However, his experiments suggest that there are values of the explanatory variable for which the conditional probability distribution is not unimodal, and a unimodal likelihood model has a bias from such a conditional probability distribution, and this bias issue may become critical in situations with a larger amount of training data. This study is motivated by the need to reduce such a bias, and develops an approximately unimodal likelihood model that is not guaranteed to be unimodal, but can represent up to a conditional probability distribution that is close to unimodal, according to the idea of the mixture model. We also investigate the effectiveness of the proposed likelihood model through numerical experiments with real-world ordinal data.
Figures/Tables
unimodal
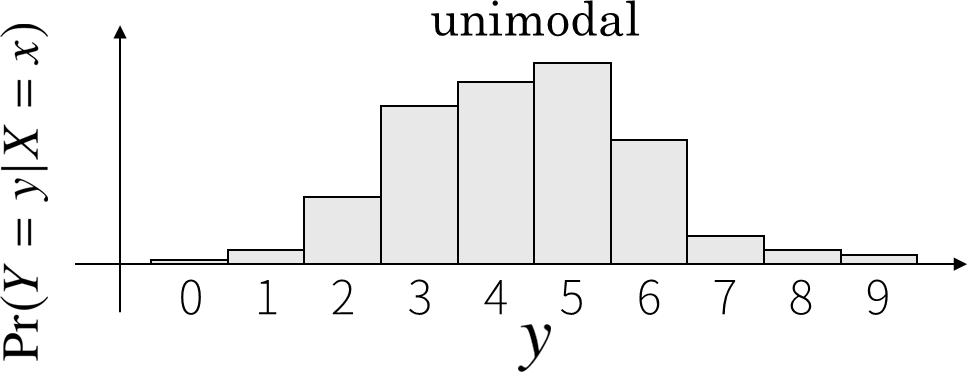
approximately unimodal
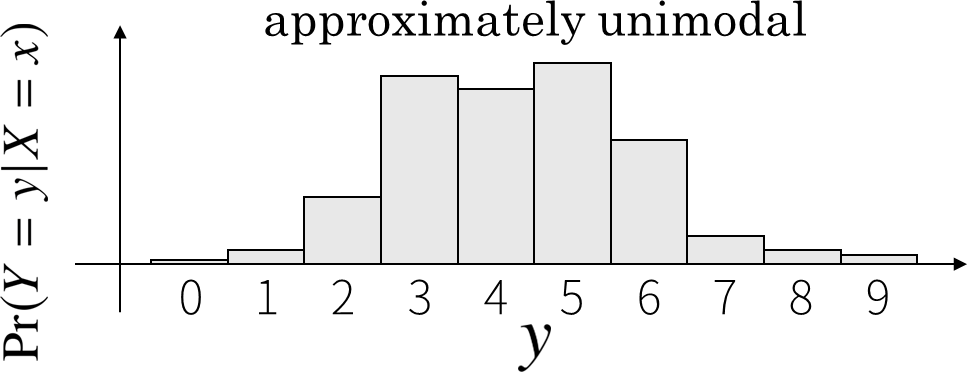