Enhancing grain futures price prediction with a multi-objective ensemble learning model using residual learning
Conference
65th ISI World Statistics Congress 2025
Format: CPS Abstract - WSC 2025
Keywords: artificial intelligence, futures prices, intelligent optimization algorithm, multi-objective,
Session: CPS 41 - Agricultural Statistics — Productivity and Crop Forecasting
Tuesday 7 October 4 p.m. - 5 p.m. (Europe/Amsterdam)
Abstract
Food security has always been a central task for governments around the world. In recent years, the world food market has experienced major fluctuations due to the COVID-19 and the Russian-Ukrainian military conflict, which have posed a major threat to the food security of some countries. Global organizations and governments should urgently take the necessary measures to maintain the stability of the world food market to avoid humanitarian crises, such as famine. Accurate forecasting of grain futures prices (GFPs) can provide key information for macroeconomic regulation of grain markets. For example, accurate forecasts of GFPs can provide information on grain supply and demand in the future. However, the price of grain futures is influenced by supply and demand, national policies, and the condition of substitutes. These factors have a highly non-linear relationship with futures prices. Therefore, GFP series are usually chaotic and non-linear, which makes accurate prediction of GFPs a highly challenging task.
In this paper, a two-stage multi-objective ensemble learning model is proposed. The ensemble learning model consists of five modules: data processing module, main prediction module, residual learning module, interval prediction module, and performance evaluation module. In this paper, we employ the complete ensemble empirical mode decomposition (CEEMD) algorithm to decompose the original GFP series and evaluate all IMFs using the sample entropy to complete the reconstruction of the GFP series. Next, we obtain preliminary forecast outputs and residuals based on the primary predictive models identified within the model pool. Following that, the preliminary predictions are adjusted by the residual forecasts derived from the residual learning module to get the final point forecasts. In addition, the introduction of intelligent interval adjustment coefficients allows the ensemble learning model to balance prediction accuracy and interval width to achieve excellent interval prediction performance. To test the performance of the proposed ensemble learning model, this paper conducts multiple experiments with three datasets: Dalian corn futures, London wheat futures, and U.S. wheat futures. The results of multiple comparison experiments show that (1) The proposed new multi-objective ensemble learning model with the residual learning module, has better forecast performance than the traditional ensemble learning framework. (2) The processing of the original futures price time series using the CEEMD algorithm and the sample entropy can effectively improve the model’s prediction accuracy. (3) The adoption of multi-objective Lichtenberg algorithm (MOLA) significantly enhances the predictive performance of the proposed ensemble learning model for grain futures price prediction. (4) The introduction of intelligent interval adjustment coefficients effectively improves the interval forecast performance of the forecasting framework. The proposed model can achieve highly accurate interval forecasting accuracy with minimal interval widths.
Figures/Tables
Flowchart of the proposed methodology
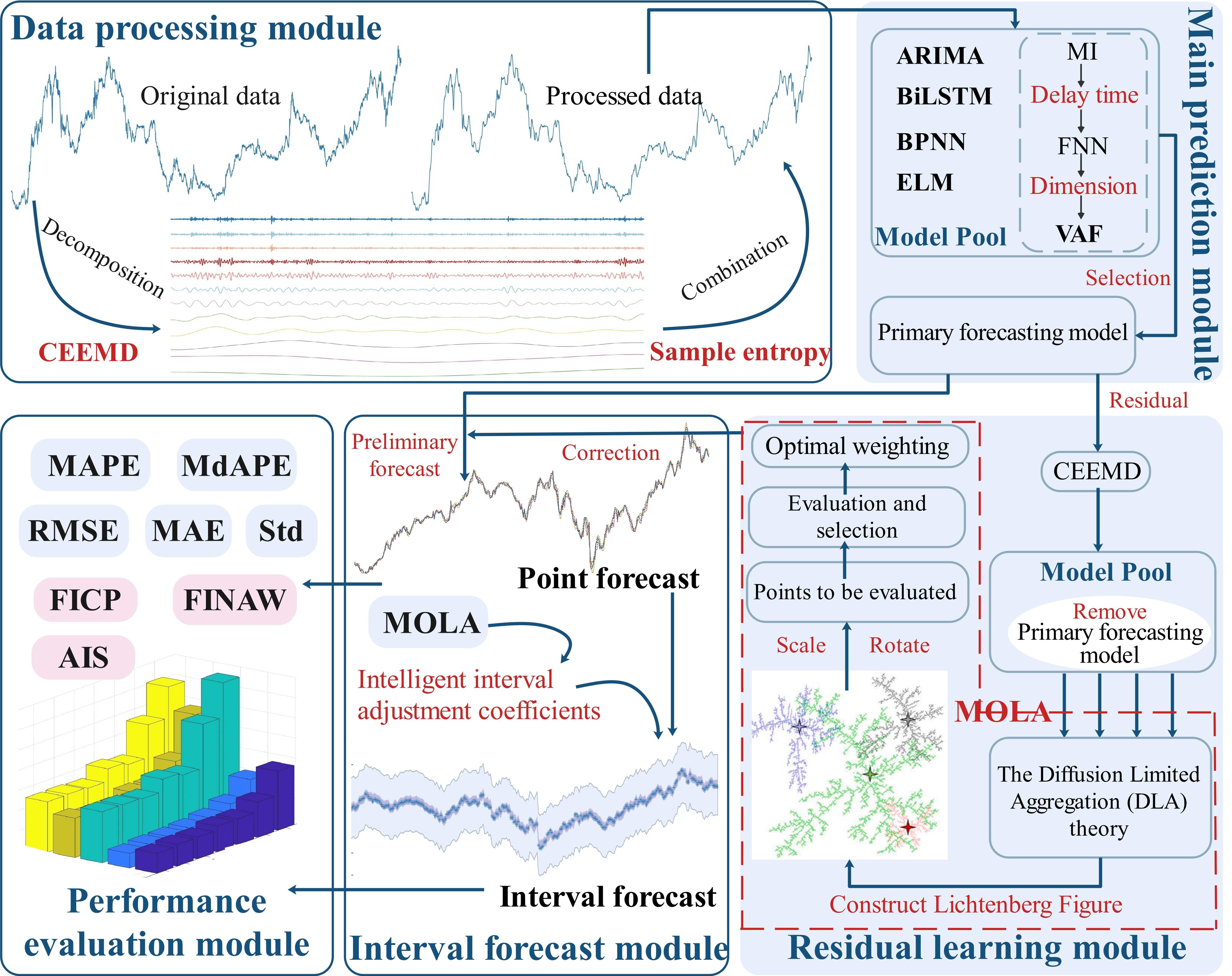
Comparison of the structure of the traditional model and the residual learning model
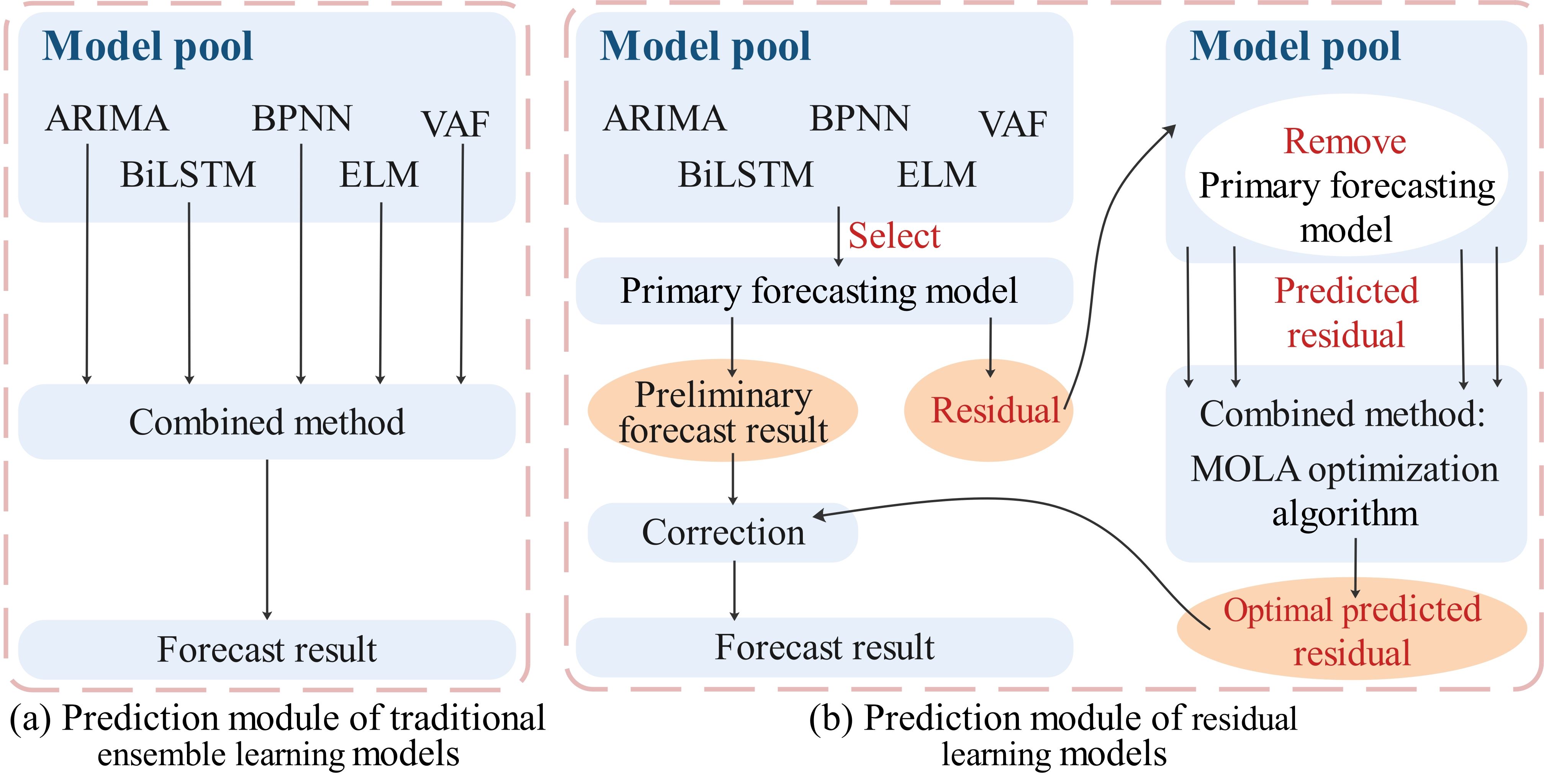
Comparison of experimental results
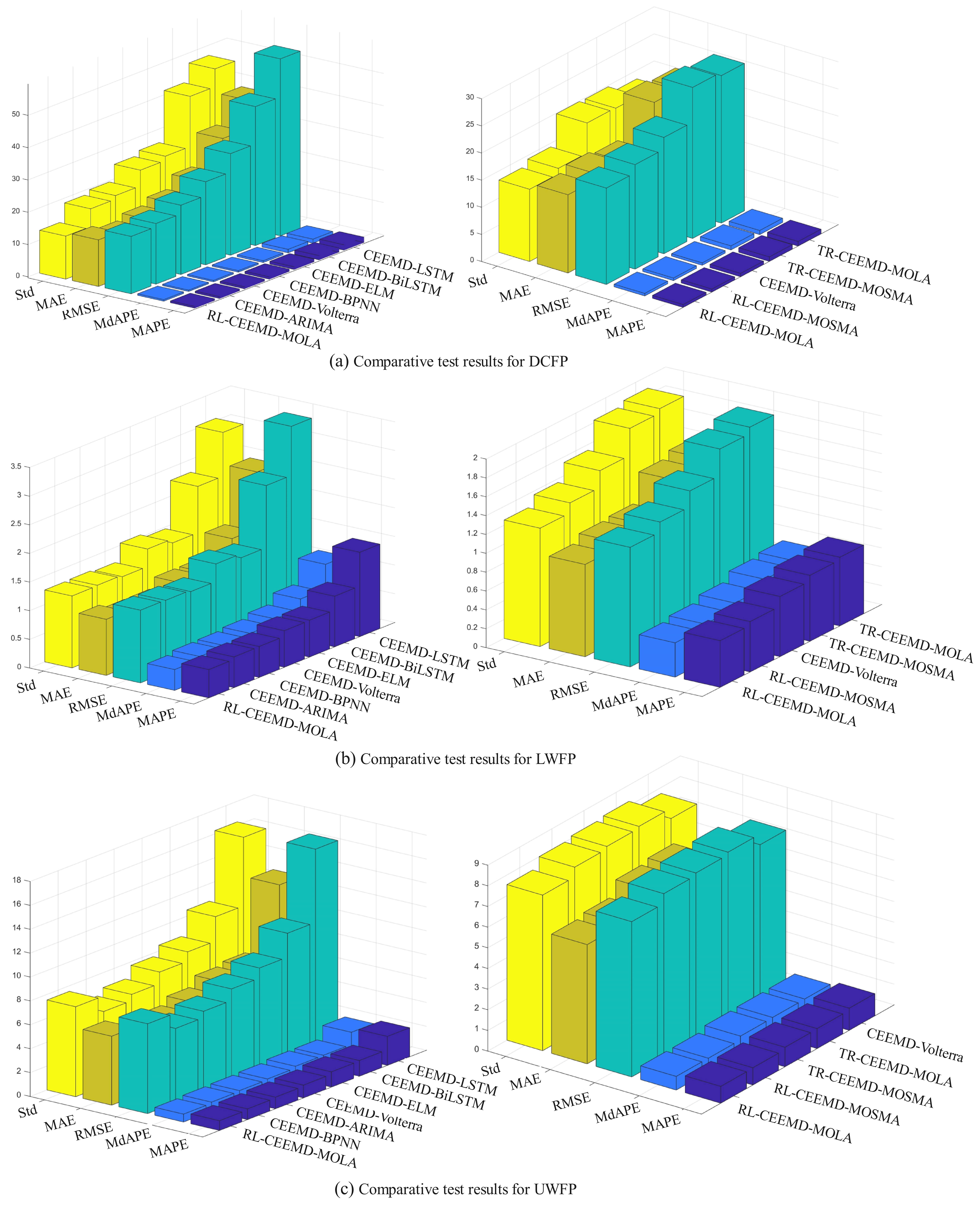