Estimating Covariance Functions for High-Dimensional Functional Time Series with Dual Factor Structures
Conference
65th ISI World Statistics Congress
Format: CPS Abstract - WSC 2025
Keywords: functional data analysis, functional time series, large dimensional covariance model
Session: CPS 10 - Factor Analysis and Structural Modelling
Tuesday 7 October 5:10 p.m. - 6:10 p.m. (Europe/Amsterdam)
Abstract
We consider high-dimensional stationary functional time series with dual functional factor model structures. A high-dimensional fully functional factor model is imposed on the observed functional processes whereas a low-dimensional one is assumed for the latent functional factors via the series approximation. Our primary interest is to estimate the large matrix of covariance functions which satisfies the so-called functional “low-rank plus sparse” structure. We extend the classic principal component analysis technique to functional time series and estimate the functional factor loadings, common factors and covariance of functional common components. A functional generalised shrinkage is subsequently applied to the estimated idiosyncratic covariance functions. Under some regularity conditions, we derive the large sample theory of the developed estimates, including the consistency of the estimated factors and functional factor loadings and the uniform convergence rates of the estimated covariance functions. Both the simulation and empirical studies are provided to demonstrate reliable finite-sample performance of the developed model and estimation methodology.
Figures/Tables
Fig_2a
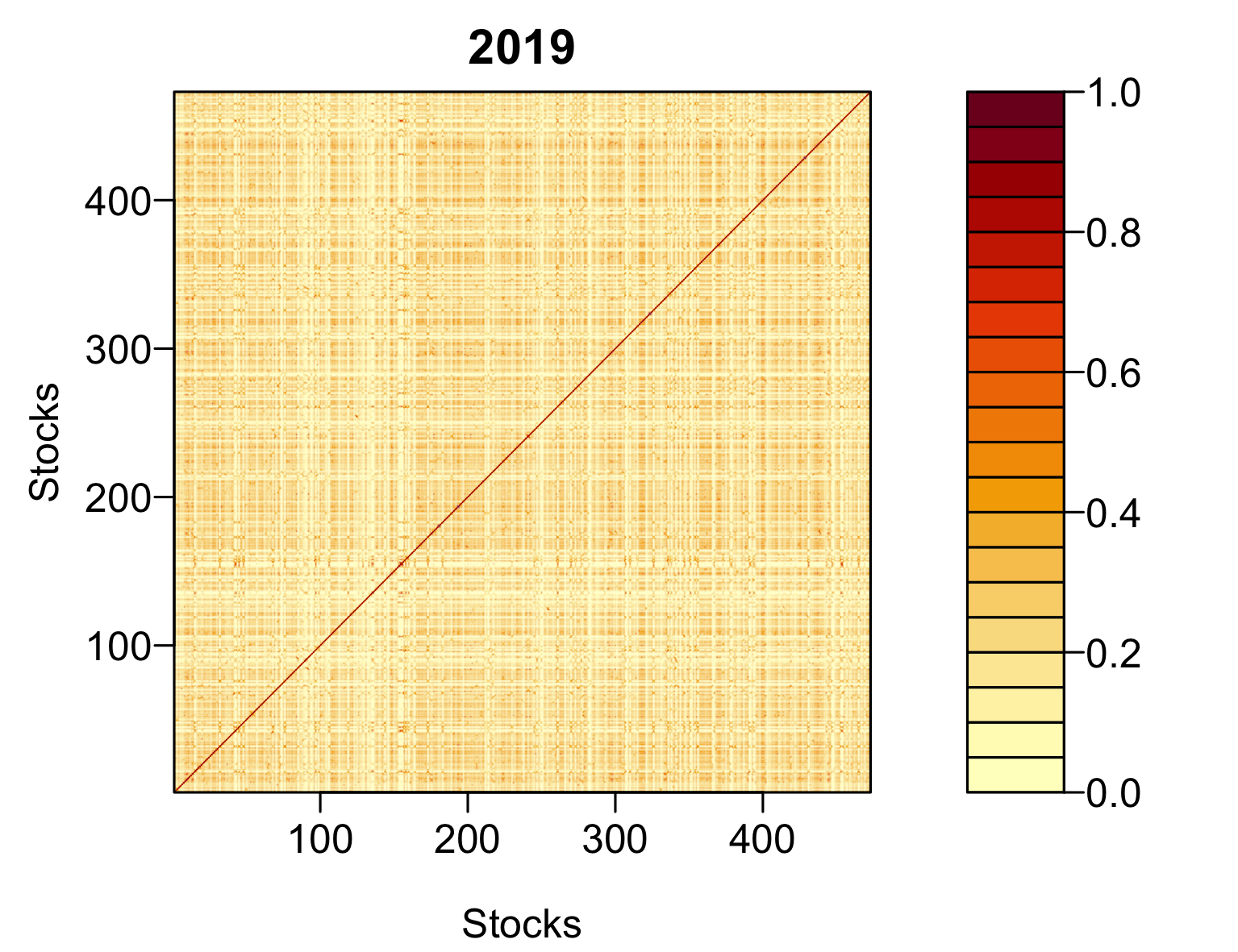
Fig_2b
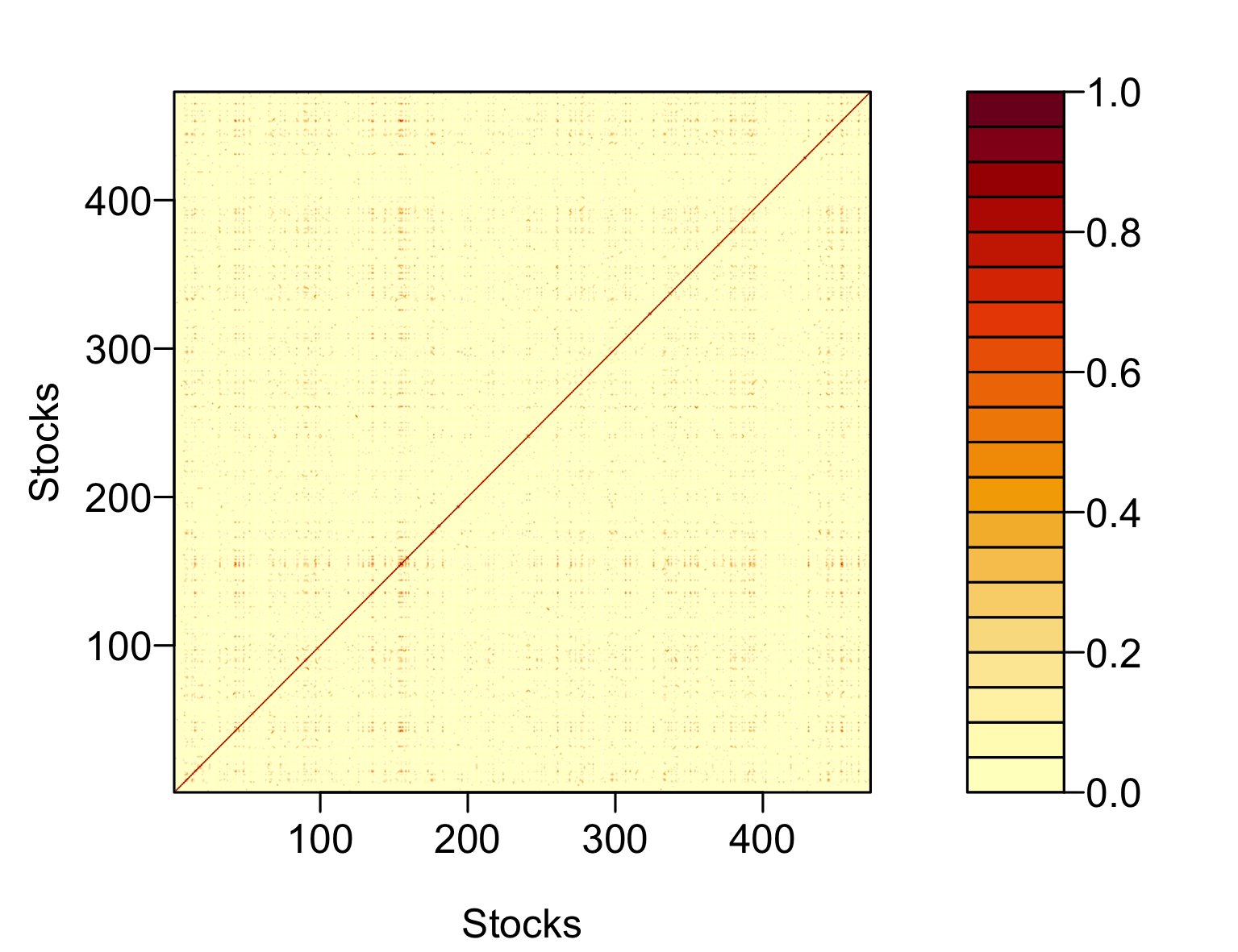
Fig_2c
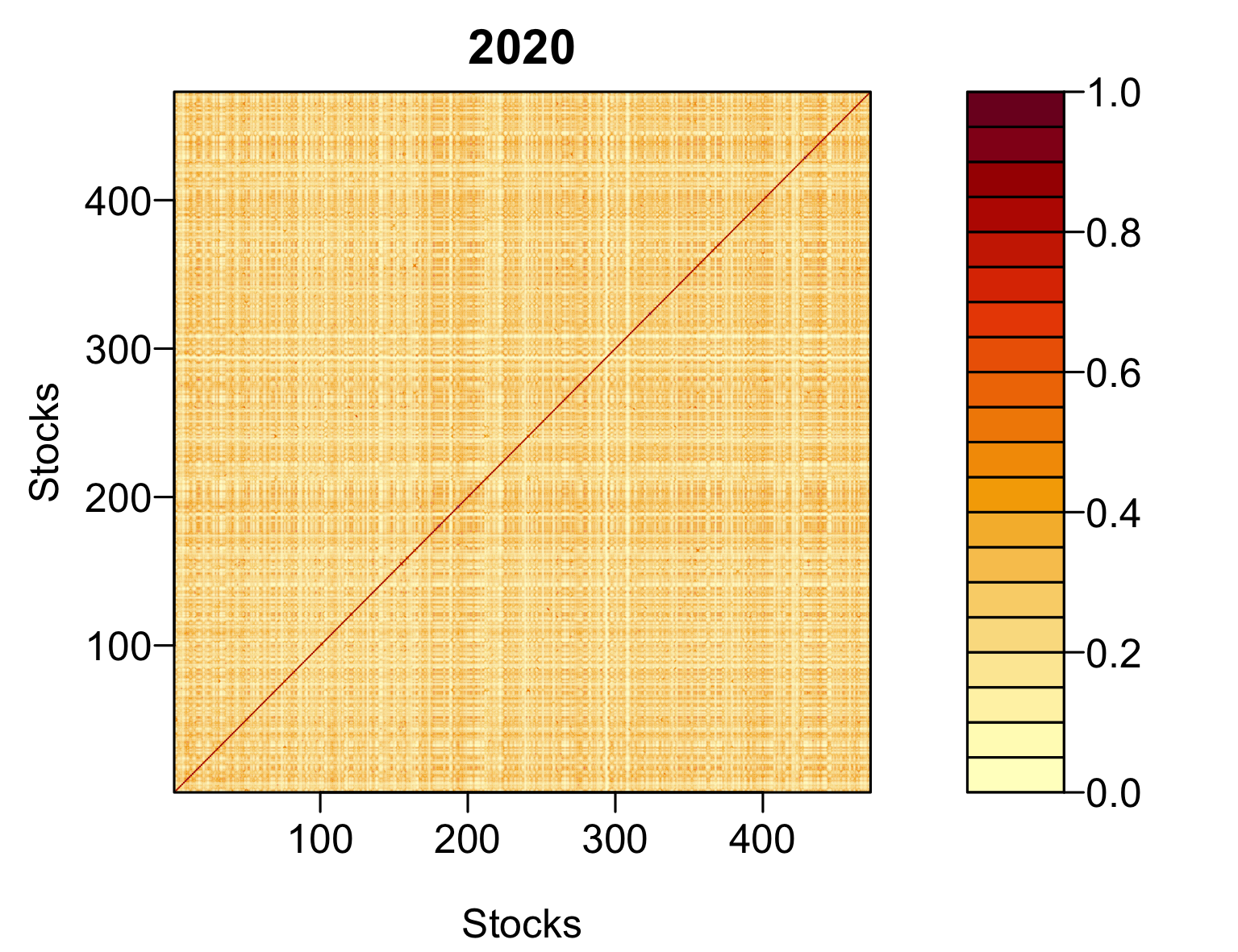
Fig_2d
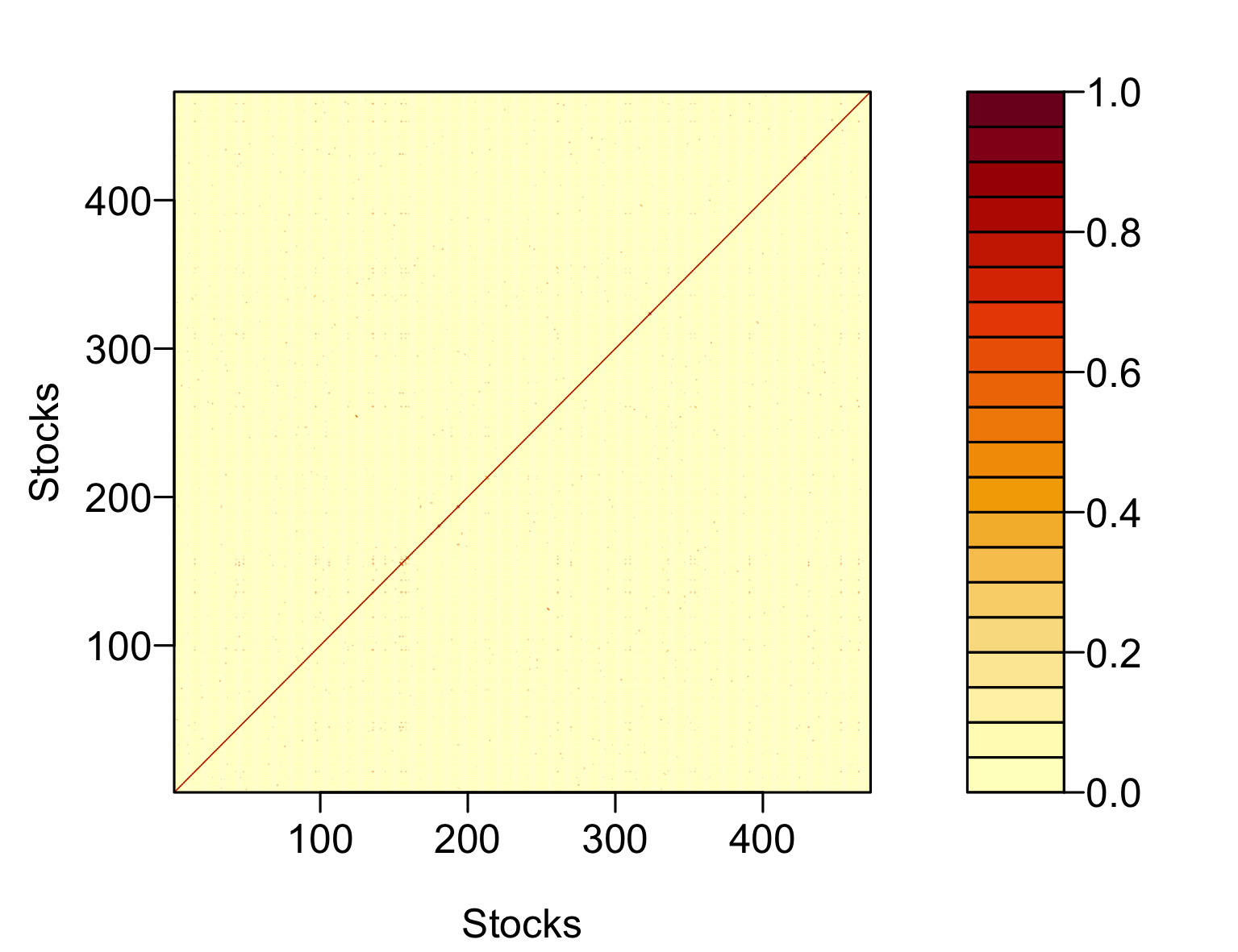
Fig_2e
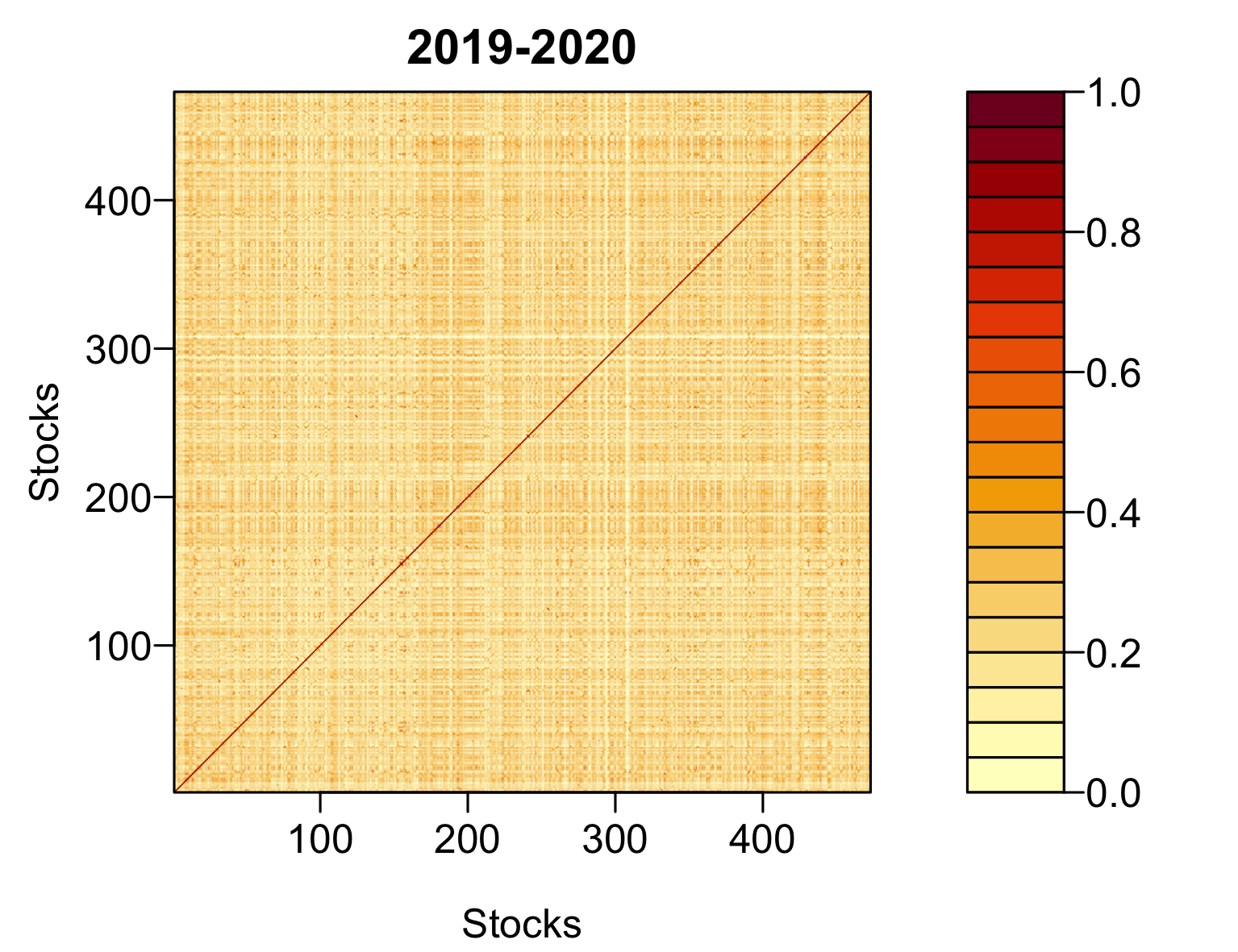
Fig_2f
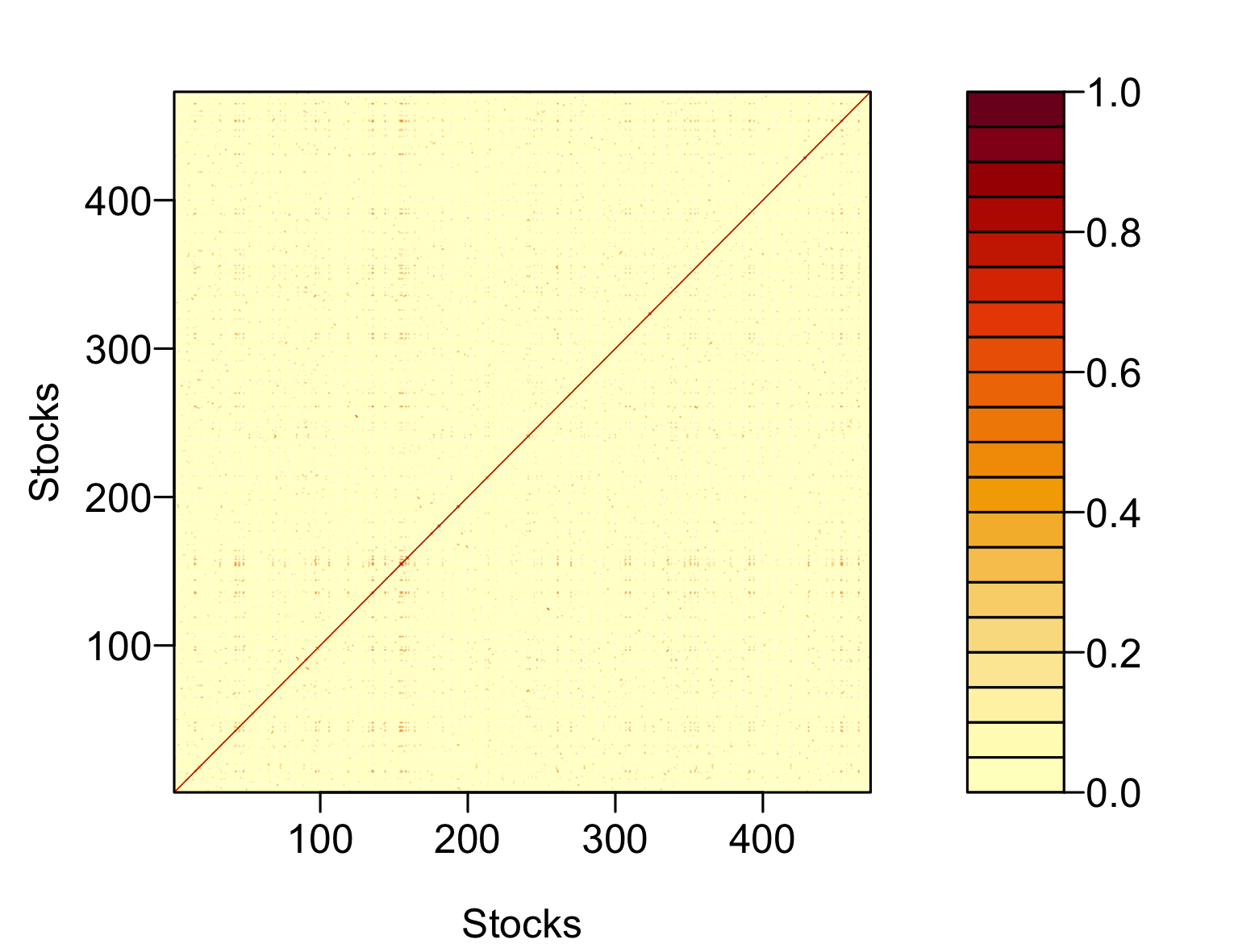