Causal Machine Learning Models for Predicting Low Birth Weight in Midwife-Led Continuity Care Intervention in North Shoa Zone, Ethiopia
Conference
65th ISI World Statistics Congress
Format: CPS Abstract - WSC 2025
Session: CPS 31 - Clinical and Public Health Research
Wednesday 8 October 4 p.m. - 5 p.m. (Europe/Amsterdam)
Abstract
Introduction: Low birth weight (LBW) is a critical global health issue that disproportionately affects infants, particularly in developing countries. This study aimed to adopt causal machine learning (CML) algorithms for predicting LBW in newborns, drawing from midwife-led continuity care.
Methods: A quasi-experimental study was carried out in the North Shoa Zone from 2019 to August-September 2020, Ethiopia. This study implemented CML to predict LBW. In this study, data preprocessing was conducted, including data cleaning. The study employed CML to find the classifier that is most appropriate for the predicting classification of LBW. Gradient boosting algorithms were applied to estimate the causal effect of midwife-led continuity care on LBW. Meta-learner algorithms were also used to estimate the individual average effect, the average treatment effects, and their performance.
Results: The study results reveal that Causal K-Nearest Neighbours was the most effective classifier based on accuracy, and estimated LBW using a 94.52% accuracy, 90.25% precision, 92.57% recall, and an F1 score of 88.2%. Meconium aspiration, perinatal mortality, pregnancy-induced hypertension, vacuum babies in need of resuscitation, and previous surgery were identified as the top five effective features affecting LBW. The estimated LBW on the causal effect between midwife-led continuity care, and other professional groups of mothers by using gradient boosting algorithms was 0.2347. The estimated average treatment effect (ATE) for S-learner was 0.284, which is lower than 0.216, the true ATE, and the estimated individual treatment effect for T and X-learner was less than -0.5, which means mothers would not have midwife-led continuity care.
Conclusions: Based on the findings, the Causal K-Nearest Neighbours is a more accurate and effective classifier based on accuracy. S-learner and R-learner models with the XGBoost Regressor and BaseSRegressor offer accurate estimations of individual treatment effects for assessing the impact of midwife-led continuity care. Promote midwife-led continuity care to stabilize LBW.
Figures/Tables
Figures1_page-0001
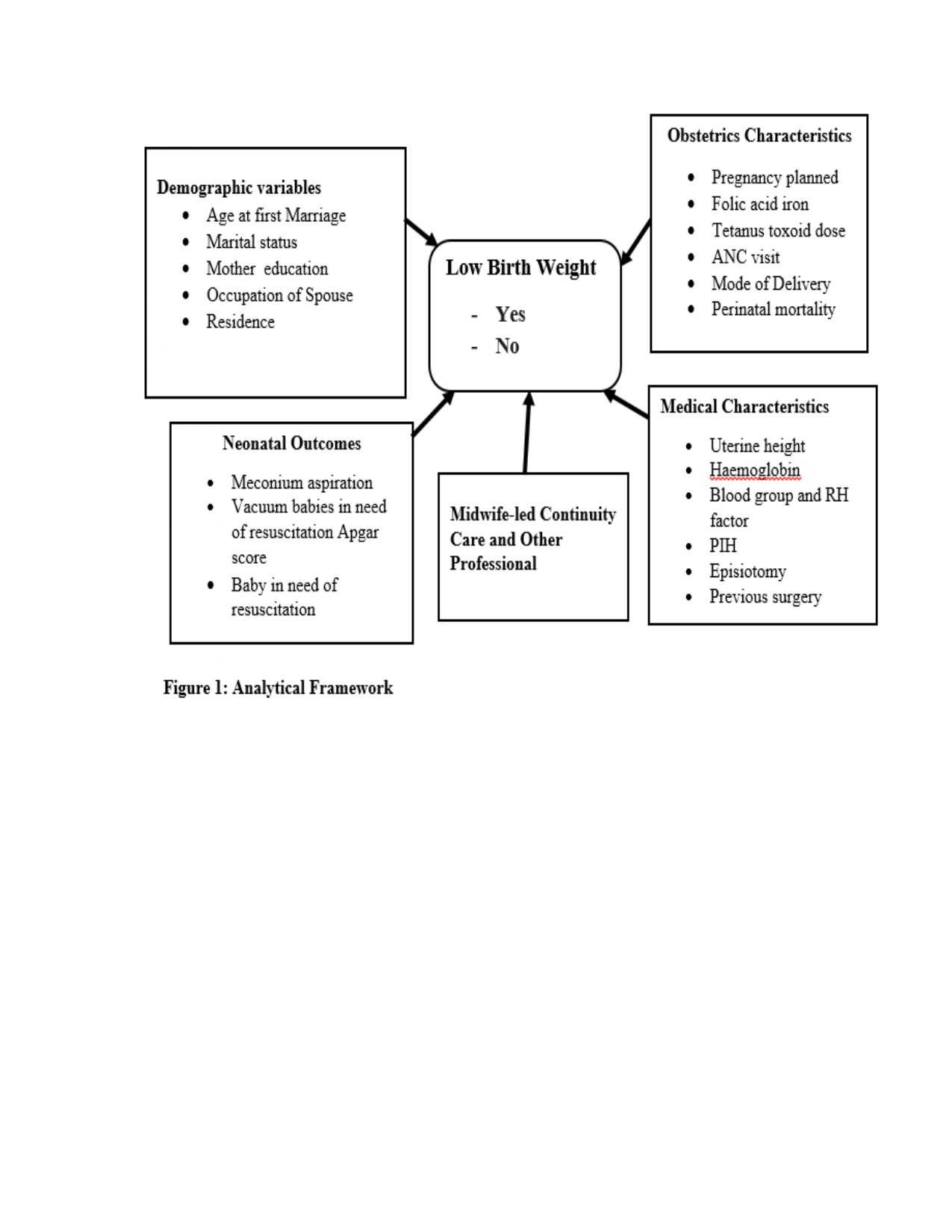
Figures1_page-0002
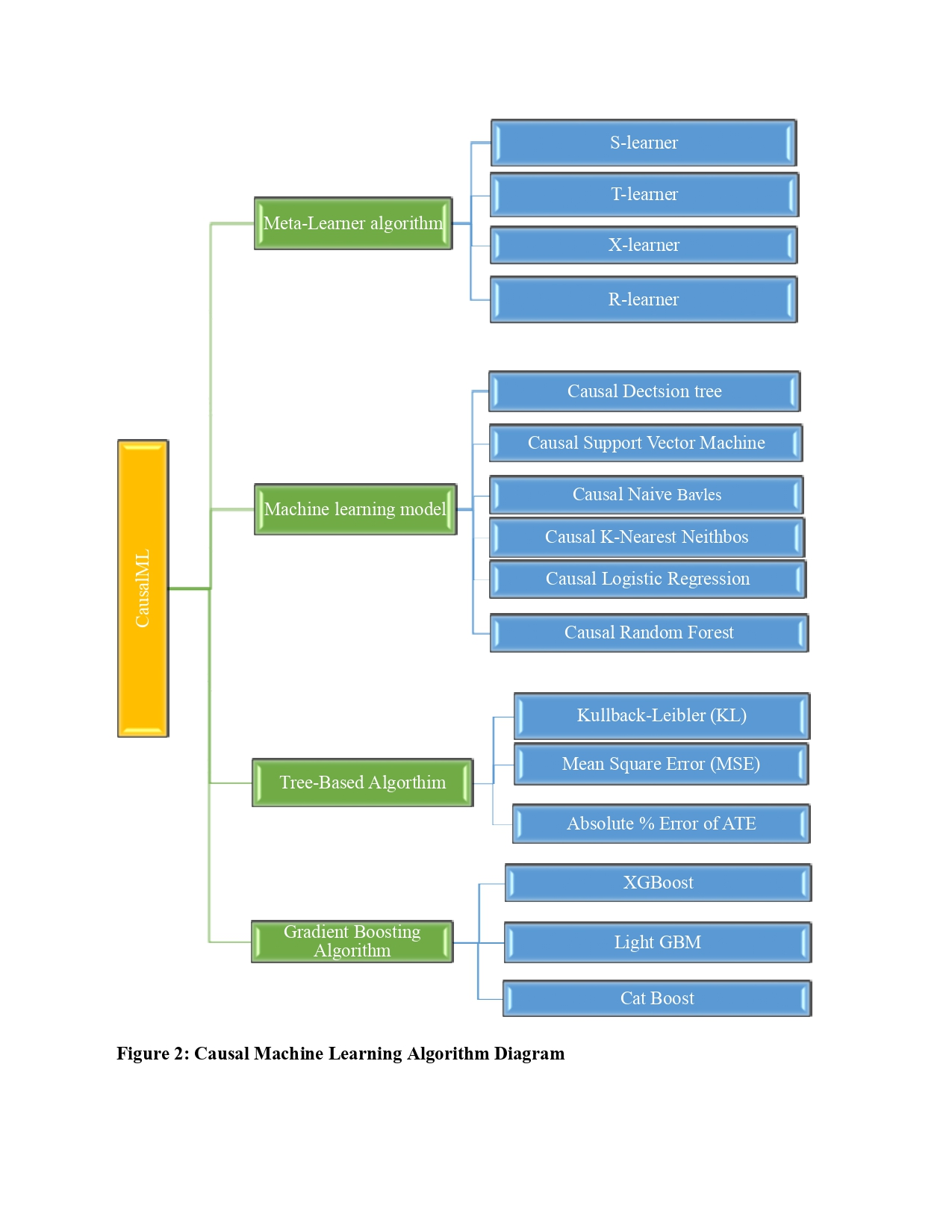
Figures1_page-0003
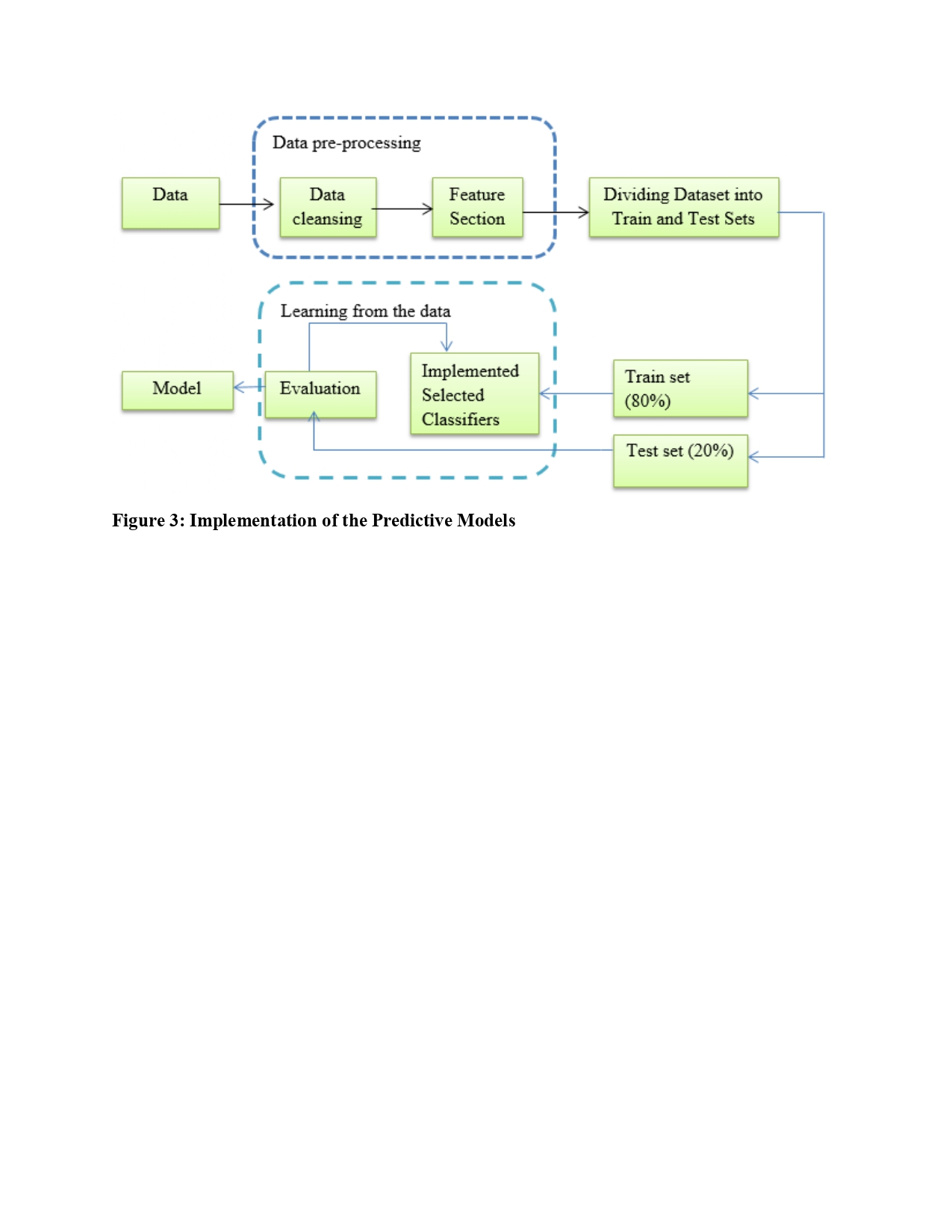
Figures1_page-0004
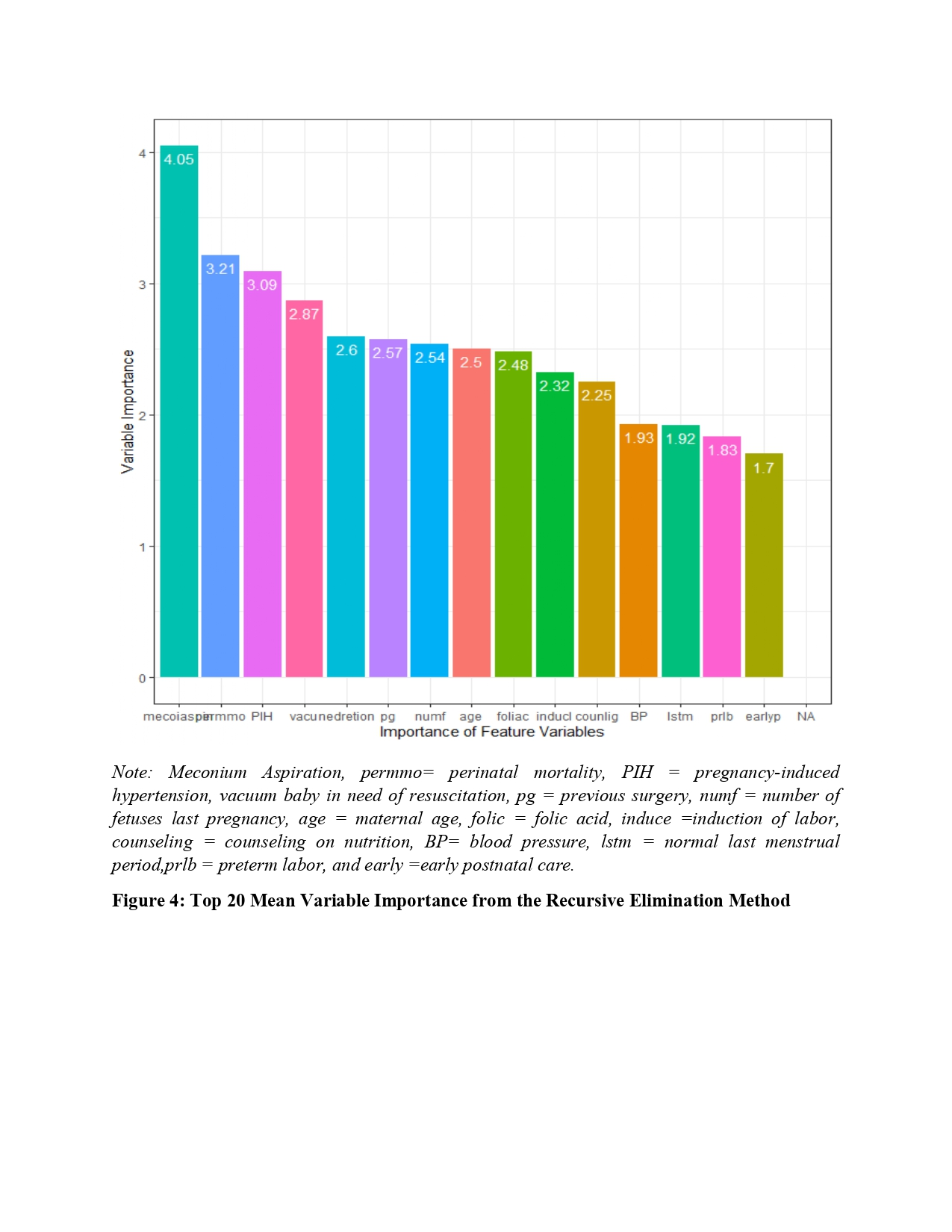
Figures1_page-0008
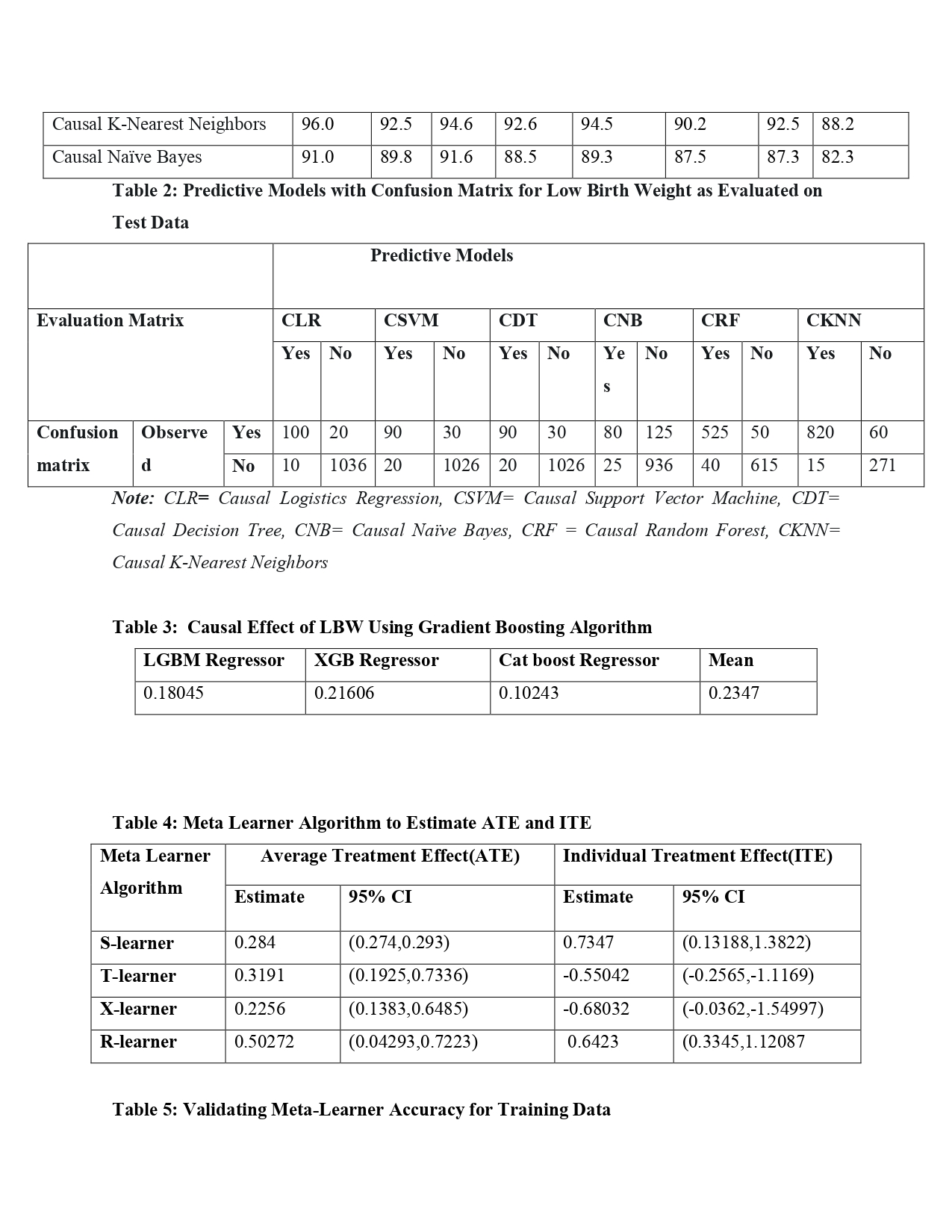
Figures1_page-0009
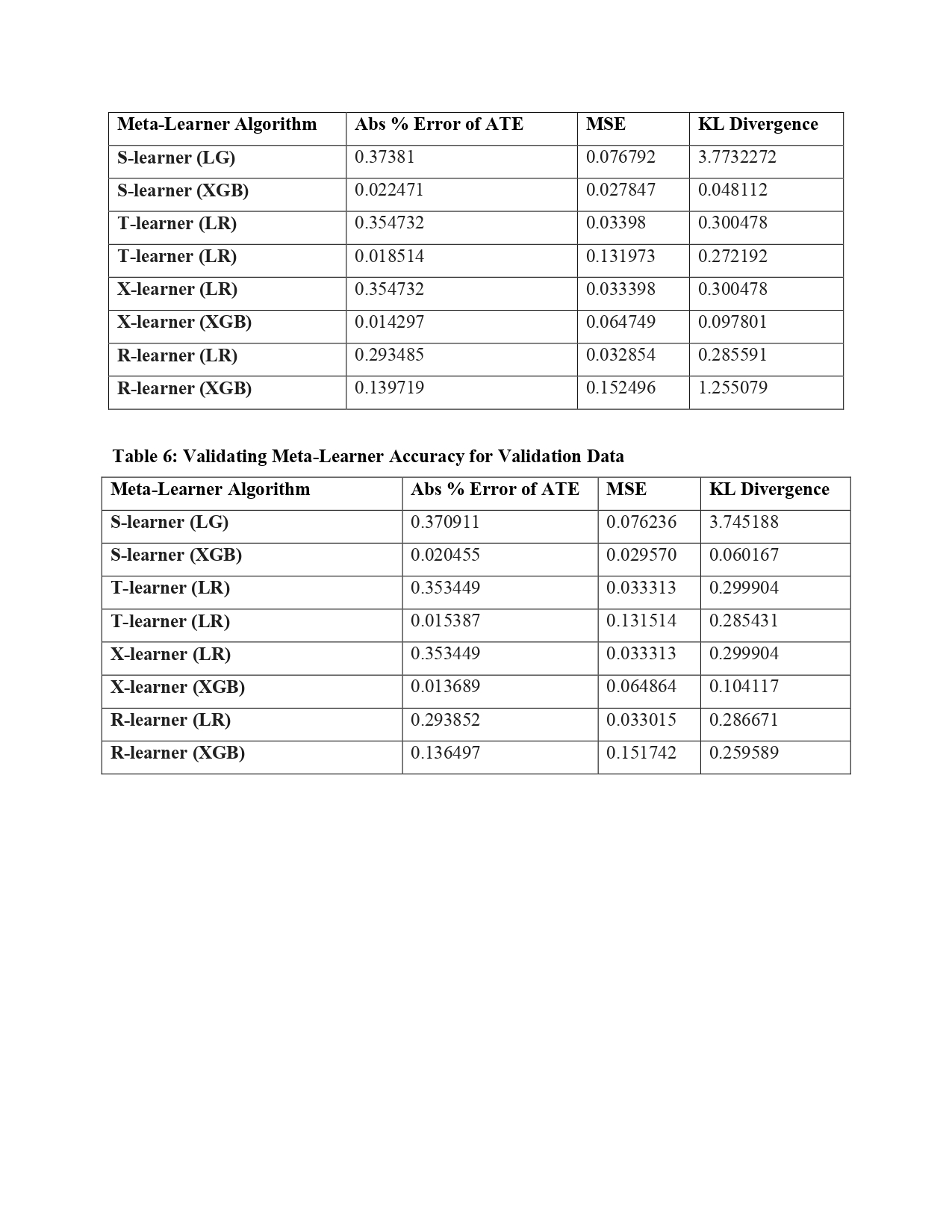